Secure AI Integration: Supercharging Your Business
Over the coming years, artificial intelligence (AI) will profoundly reshape the business landscape, challenging traditional paradigms and rewarding innovation. Companies that integrate AI strategically will gain a distinct competitive edge, much like the early adopters of the nascent Web did decades ago. AI represents not just an opportunity but a necessity, so businesses must embrace it responsibly and purposefully.
When integrated strategically, AI has the potential to revolutionize every facet of an organization. It can enable businesses to create and deliver innovative, differentiating services or products that set them apart from competitors. AI can facilitate expansion into new markets or uncover novel opportunities in niche segments. Beyond innovation, AI can optimize existing processes, resulting in more efficient operations, improved product or service quality, and greater customer satisfaction. By harnessing AI with a clear vision, organizations can unlock unprecedented value and establish themselves as industry leaders.
To fully realize the potential of an AI-powered business, organizations must adopt a strategic, enterprise-wide approach, aligning AI initiatives with overarching business objectives. The leadership must develop a strategic rationale for AI adoption and a clear value proposition by mapping AI initiatives to strategic goals and key performance indicators (KPIs). This rationale ensures organizational support and increases the likelihood of delivering on AI's promise. By aligning with business objectives, stakeholders can focus on tangible outcomes that deliver the strategic objectives. Of course, this AI adoption requires careful security considerations and a holistic security framework.
Secure AI
The shift towards AI-enabled applications increases complexity and opens new avenues for cyber attacks. In addition to traditional cyberattacks, organizations must defend AI-enabled systems against AI-specific attacks. To enhance the security of AI systems, organizations must integrate comprehensive security frameworks and adhere to best practice recommendations.
AI-Specific Attack Vectors
AI systems are still subject to traditional cyberattacks, such as social engineering, vulnerability exploits, and credential theft. Therefore, traditional security practices, such as access control, vulnerability management, intrusion detection, and encryption are vital. However, security teams must also understand attack vectors specific to AI applications.
Data and model poisoning attacks alter the data or model to compromise the model's integrity. Attackers will typically use one of three common types of data poisoning attacks. First is the targeted insertion of adversarial samples into the training data. Next is non-targeted sample insertion, where the goal is typically to disrupt the model. Finally, the attacker may seek to modify existing samples by changing labels or features. In model poisoning, the attacker targets the model and its parameters.
Interconnected AI agents communicate and collaborate to achieve their objectives. However, the risks associated with unauthorized agent-to-agent communication are substantial. Unauthorized interactions among AI agents can lead to unintended data sharing, the spread of malicious code, and the execution of unintended or harmful operations. Also, agents with update privileges could corrupt or destroy the data. The autonomous nature of agentic AL magnifies this risk.
Prompt injection manipulates AI prompts in a way that changes the agent's behavior to execute unintended actions. Risks include context leakage and providing attackers with unauthorized access to sensitive internal documents.
When using third-party deep learning models, these models could have model backdoors hidden within them. Therefore, organizations should use trusted models from trusted sources.
An exploratory attack collects information about the training data and the model during inference. Exploratory attacks can duplicate the model or act as a precursor to a privacy attack. The attacker uses reversing techniques to discover how the algorithms work. Privacy attacks seek to gain information about the data used to train the model. These attacks target the production system, typically through API access. Privacy attacks may seek to determine whether specific data was used in training and infer membership. Another privacy attack seeks to reconstruct training data about a given instance or the full training data set.
Building Secure AI Systems
Security by Design: A secure-by-design approach evaluates the potential dangers of AI models and builds these systems with security considerations from the outset. Development teams and security professionals must understand the threats targeting each phase of developing, deploying, and operating AI systems.
Data Security: Data security focuses on data confidentiality, integrity, and availability throughout its lifecycle. Organizations should practice data minimization, ensuring they collect and use only the data necessary for specific AI applications, reducing the risk of breaches. Data not needed to train the model, especially privacy-related data, should be removed before training. They should also anonymize data used for AI training or generate synthetic data without personal information to mimic actual data, where appropriate. Finally, data should be encrypted when in use and at rest.
Data Provenance and Lineage: Data provenance and data lineage are critical topics for AI applications. Data provenance focuses on the origin and history of the data, documenting where it came from, how it was created, who created it, and how it has been modified. Recent advances in data provenance include using blockchain technology to track the data history. Documented data provenance helps ensure the data's quality and integrity and is essential to regulatory compliance, such as GDPR and CCPA. Data lineage applies version and change control to the data and respective model through collection, preparation, training, testing, and retraining.
Secure Agent-to-Agent Communication: Multi-agent AI systems have multiple specialized agents working together to solve intricate challenges like a well-coordinated team. The design of Agentic AI systems allows them to handle complex problem-solving, decision-making, and operational management tasks. However, the agent-to-agent communications provide another attack vector for adversaries. Therefore, the communication protocols between agents must be secure, and AI agents must incorporate the concept of least privilege.
AI Testing and Monitoring
The AI system and environment should undergo all the traditional testing, such as performance and penetration testing. However, testing methods and considerations unique to AI systems are described below. Organizations must employ a comprehensive testing methodology to ensure the performance, resilience, and integrity of AI models, data pipelines, and hosting environments. Furthermore, organizations should monitor and conduct regular audits to identify vulnerabilities early, detect issues with model performance, and ensure compliance with regulations and industry standards, such as the EU AI Act, GDPR, PCI-DSS, and HIPAA.
Quality Testing: Quality testing focuses on ensuring the AI model achieves the expected results and solves the problem for which it was designed. During training, the developers review statistical results to determine if the model achieves the desired results from the training data. The same tests are repeated during the testing phase to ensure the results remain acceptable when processing previously unseen data and that the model does not over-fit the training data.
Adversarial Testing: In adversarial testing, the security team proactively attempts to exploit vulnerabilities in the AI process, much like red teaming or penetration testing used for securing traditional software development. Adversarial AL is built on three pillars: a) recognize training and inference stage vulnerabilities, b) develop corresponding attacks, and c) devise countermeasures.
Model Drift Monitoring: Drift detection, including concept and data drift, is crucial to model monitoring. Model drift causes performance degradation due to changes in the underlying data distribution. Concept drift occurs when the statistical properties of the target variable change over time, while data drift happens when the distribution of input data changes. Implementing robust drift detection mechanisms allows organizations to identify when models must be retrained or updated, ensuring that AI systems remain accurate and relevant as data patterns or business conditions. Advanced monitoring systems can provide real-time alerts or automatically trigger retraining when performance metrics fall below the defined thresholds.
Model Behavior Monitoring: Model behavior monitoring analyzes the model to detect unusual patterns using anomaly detection algorithms. These algorithms detect deviations from normal patterns, which could indicate attacks, breaches, or drift. These systems can alert response teams in real-time, enabling quick threat mitigation.
LLM Security
LLM firewalls use real-time input and output scanners to detect security risks, including adversarial prompt attacks, sensitive data leakage, and integrity attacks. LLM firewalls can be classified as prompt, retrieval, or response firewalls. LLM prompt firewalls filter out potentially malicious prompts or redact sensitive information. To prevent sensitive data exposure and poisoning, retrieval firewalls monitor and control data during the retrieval augmented generation (RAG) stage. LLM response firewalls monitor the responses generated by the LLM to ensure they do not violate security, privacy, compliance, or ethical guidelines. Response firewalls can redact sensitive data, filter hallucinatory responses, block toxic content, and filter prohibited topics.
Input validation and filtering (IVF) checks for unauthorized commands, malicious content, adversarial input, and anomalies in the prompt and associated data. Adversarial input detection analyzes input patterns to uncover disguised threats that could cause the model to output incorrect or manipulated results. Effective IVF can prevent injection attacks and harmful instructions from compromising the model and significantly reduces vulnerabilities associated with manipulated or incorrect data.
Bias detection identifies inherent biases within LLMs that could skew outputs. Of course, not all biases are harmful, so it is important to analyze them to determine if they are unwanted. Organizations can take corrective actions when unwanted biased patterns are recognized, including adjusting algorithm parameters or retraining the model with more balanced data.
Share this
You May Also Like
These Related Stories
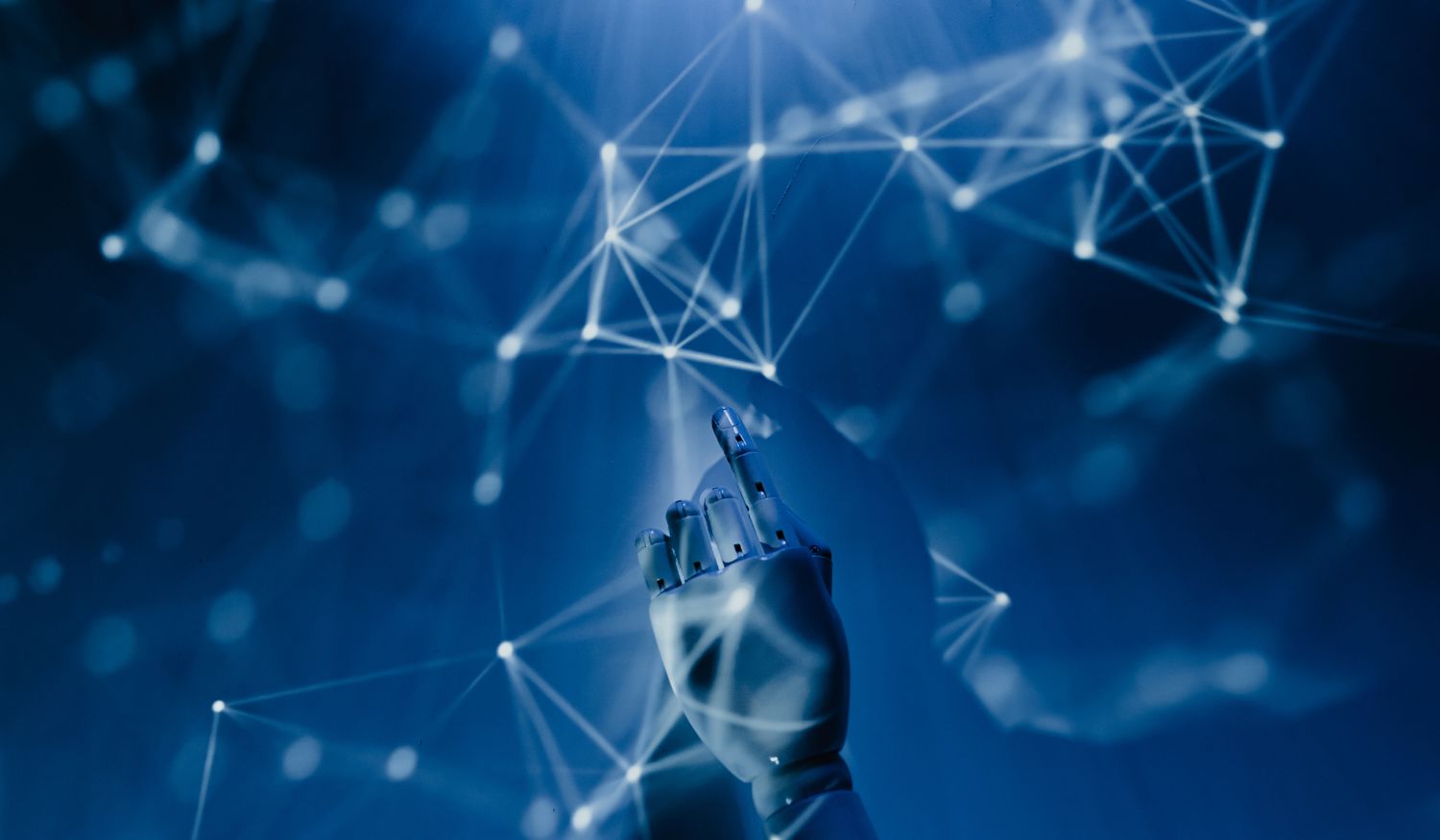
Opportunities & Risks in AI: What Every CISO Should Know
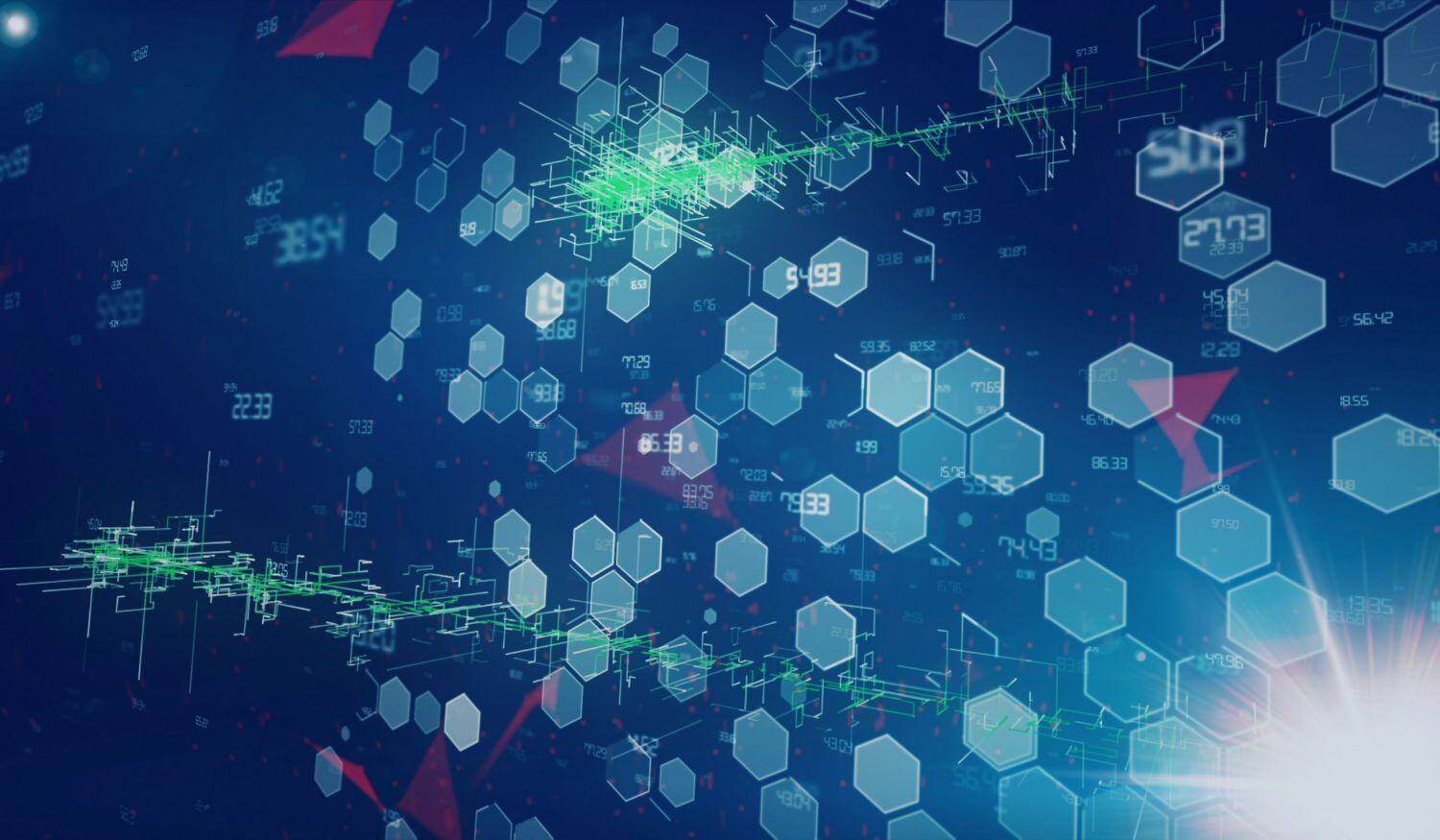
Can AI Stop Cybercriminals from Hiding Behind Cryptocurrency?
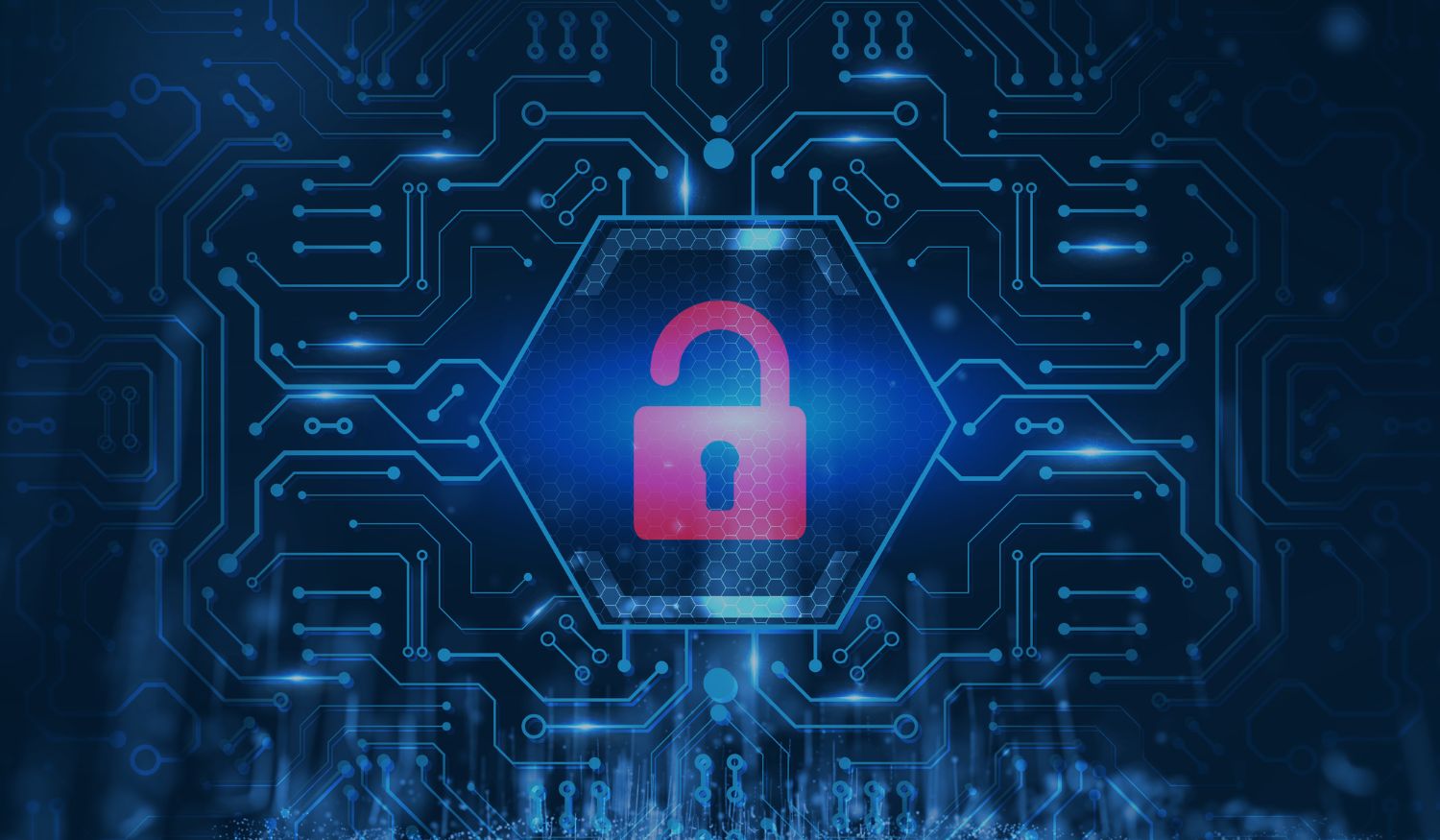