AI Adoption: Protecting Loss Of Sensitive Data
Most organizations now have Generative AI embedded across business functions from marketing and sales to engineering and legal operations. Therefore, as security professionals, our job is no longer to decide whether AI will be adopted, but to ensure it’s done responsibly. That means mitigating real risks without stifling innovation.
I’ve spent the past two years working closely with cybersecurity leaders across industries to understand their AI usage and the associated risks. What’s clear is that AI adoption has become a data protection problem at its core. Most of the risk is concentrated around what employees are feeding into AI systems, where that data is going, and whether those actions comply with the organization's obligations and tolerances.
Data Exposure Through Generative AI
There is a trade-off for CISOs, as boards are pushing for rapid innovation to remain competitive. On the other hand, CISOs and compliance teams are tasked with ensuring sensitive data doesn’t leak into unsecured or inappropriate tools.
The surface area is immense as on average there are 254 different AI applications in use per organization. While the headlines focus on tools like ChatGPT or Microsoft Copilot, there’s an expansive ecosystem of generative AI apps, everything from presentation generators to code assistants, spanning thousands of vendors globally.
Among the most pressing concerns we track:
- Sensitive corporate data in prompts: Roughly 7% of prompts contain sensitive information, including financials, IP, or legal data.
- Use of risky platforms: Approximately 6% of employees are interacting with AI tools hosted in jurisdictions with elevated compliance concerns, such as China.
- Personal account use. The majority of AI use is unsanctioned, with employees using free, personal accounts in 45.4% of cases. These are more likely to train on your data and you have fewer controls over how the data is stored.
- Lack of visibility: Security teams often have little insight into which tools are in use or what data is being submitted.
- Insufficient controls: Traditional DLP tools and firewall-based approaches are poorly suited for AI-specific use cases, especially at the prompt level.
The Failure of Legacy Controls
Existing controls, from policy documentation to traditional DLP, have struggled to adapt. Writing a policy is a common first step, and most organizations now have an AI use policy in place. However, policies alone don’t stop data loss. They don’t tell you who is using what tools, or what data is leaving the organization.
Likewise, legacy DLP technology was never built for this kind of data flow. These tools often focus narrowly on credit card numbers or PII and are based on brittle regular expression based rules that generate too many false positives. Meanwhile, labeling all sensitive data via data classification projects is slow, expensive, and often fails to cover legacy files and edge cases.
The net effect is that security teams are left either in the dark or overwhelmed, while employees continue using whatever AI tools help them get their jobs done faster.
Reframing the Role of the Security Team
Rather than being gatekeepers, security teams can lead AI governance efforts across the business. The role isn’t to block tools, but to coach the organization on safe usage, empower secure adoption, and implement governance mechanisms that scale.
Here's how we see leading organizations approaching this challenge:
- Build cross-functional governance: A steering committee representing security, legal, data, and business teams should align on risk tolerances and acceptable AI usage. This lays the foundation for a common language and priorities.
- Establish meaningful visibility: Go beyond domain-level telemetry. You need prompt-level insights into what data is being shared, with which tools, and by whom. This is what enables productive conversations with the business about risk and alternatives.
- Tailor controls to the business: Not all AI apps are created equal, and not all teams have the same risk profile. A nuanced approach means allowing some departments to use override functions while enforcing stricter rules in areas like engineering or legal. You should be able to explain to a marketing employee why a certain prompt presents risk and offer safer alternatives in real time.
- Coach rather than block: Human-centered interventions outperform blanket restrictions. If you detect a risk, explain it in context and suggest a course correction. Empower the user to remain productive while protecting the business.
- Automate enforcement with precision: This is where small language models trained specifically for data protection come in. Unlike generic LLMs or regex-heavy DLP systems, these models can recognize domain-specific sensitive data, such as M&A documents, legal contracts, or proprietary code, and enforce policy with minimal false positives.
The Broader Implications for Security Strategy
The growth of generative AI presents an opportunity for security teams to rethink their strategic role. With the right tools and mindset, security becomes a key enabler of transformation, not a barrier.
But achieving this requires a shift in approach. We can’t rely on outdated DLP systems or one-size-fits-all policies. Instead, we need to meet employees where they are: in the browser, using hundreds of different tools, moving fast, and working with sensitive data every day.
Security leaders who can offer governance frameworks that are lightweight, transparent, and effective will earn trust from their peers in the business. And they will position their teams as strategic partners in AI adoption, not just risk mitigators.
For those looking to take the first step, start by monitoring AI activity before enforcing controls. Understanding where your data is going and which teams are using which tools gives you the leverage to act strategically. Then, introduce controls incrementally, in ways that balance protection with productivity. Both your users and board will thank you.
How Harmonic Fits In
Harmonic is designed to address these exact needs. We’re a browser-based extension that deploys in under 30 minutes. Once installed, we provide security teams with immediate, granular insight into AI usage across the organization, but more importantly, we keep the load off security teams by addressing the end user at the point of data loss.
We’ve trained 21 distinct small language models that understand context-specific sensitivity across industries. This includes models for financial data, insurance claims, legal communications, engineering documents, and more. These models operate in real-time at the point of interaction.
If a user attempts to input sensitive information into a tool that’s out of policy, Harmonic intervenes in-line. We focus on coaching; we want to support and encourage adoption but in a safe and secure way. A customizable modal is displayed, branded to your company, that explains the issue and provides next steps. Depending on your settings, users can override with justification, redact data, or be redirected to approved platforms.
This allows for a far more intelligent and adaptive control experience. Security teams can enforce policies without becoming a bottleneck, and users can stay productive while staying compliant.
Recently we had a client at 90 days show a 72% reduction in data loss AND a 300% increase in adoption of AI tools. This is the future as we see it, adopt and innovate in a safe and secure environment.
Share this
You May Also Like
These Related Stories
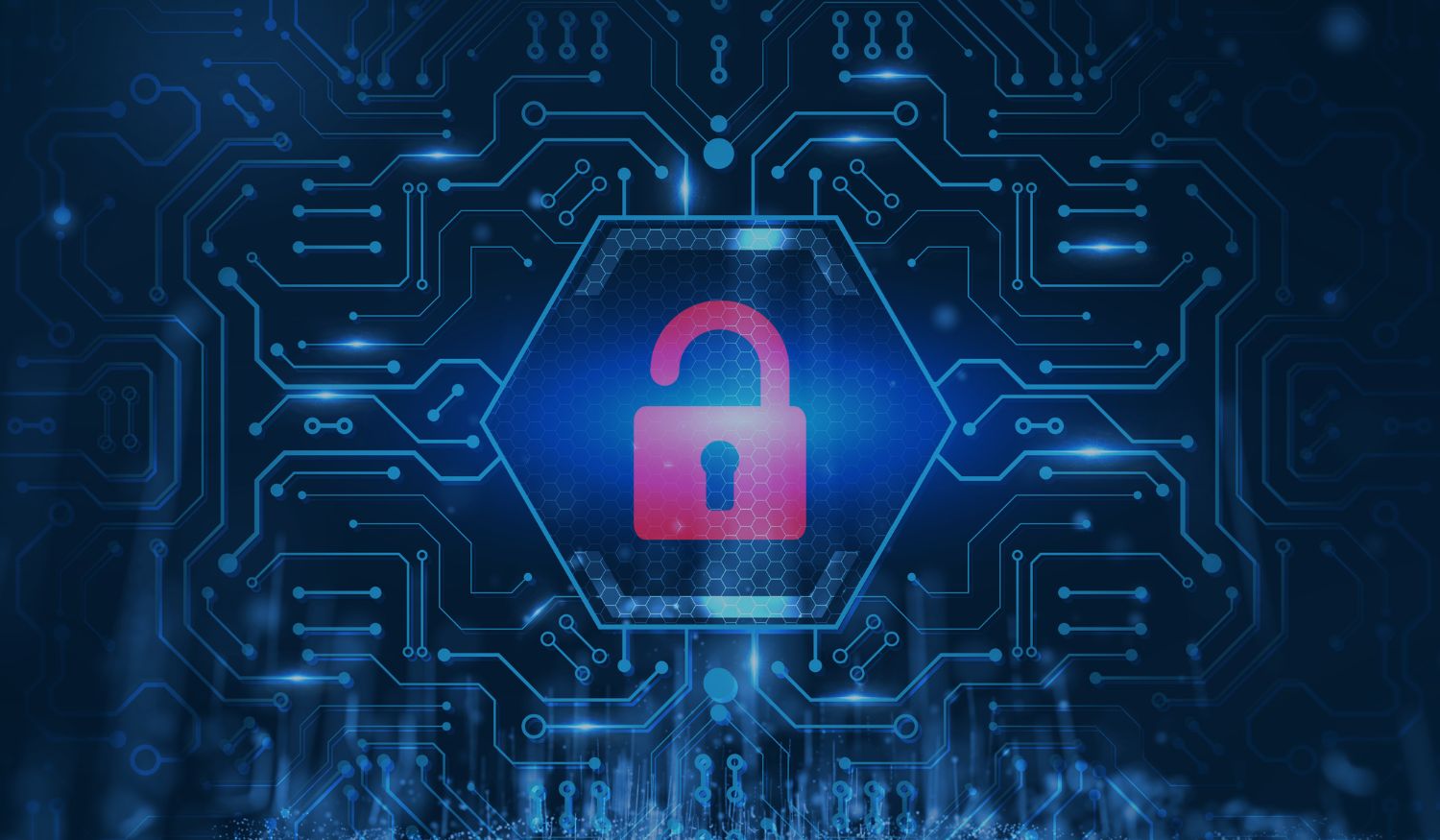
Industry Experts Share DSPM, Automation and AI Data Security Insights
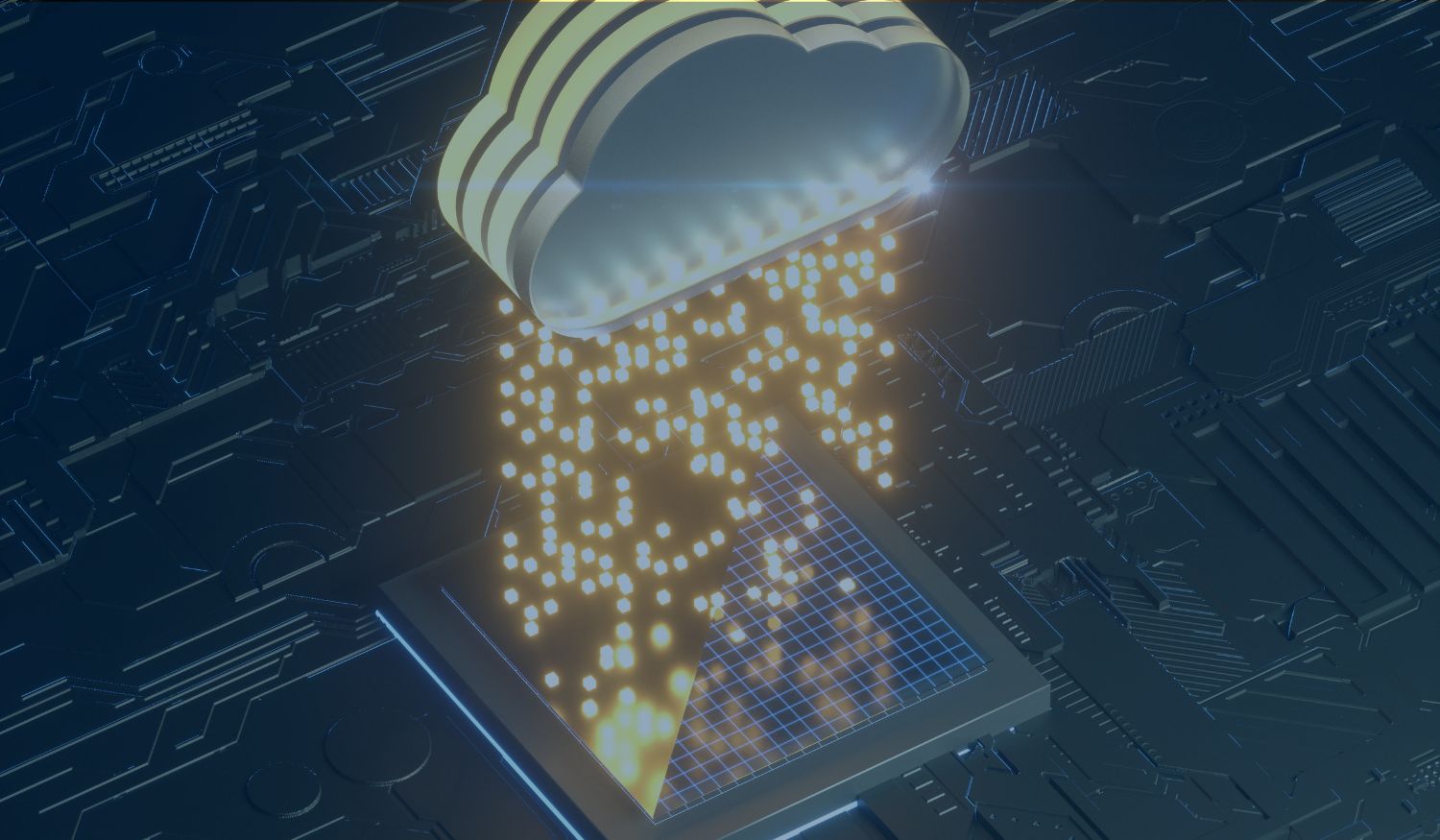
7 Considerations When Implementing Cloud Security Architecture
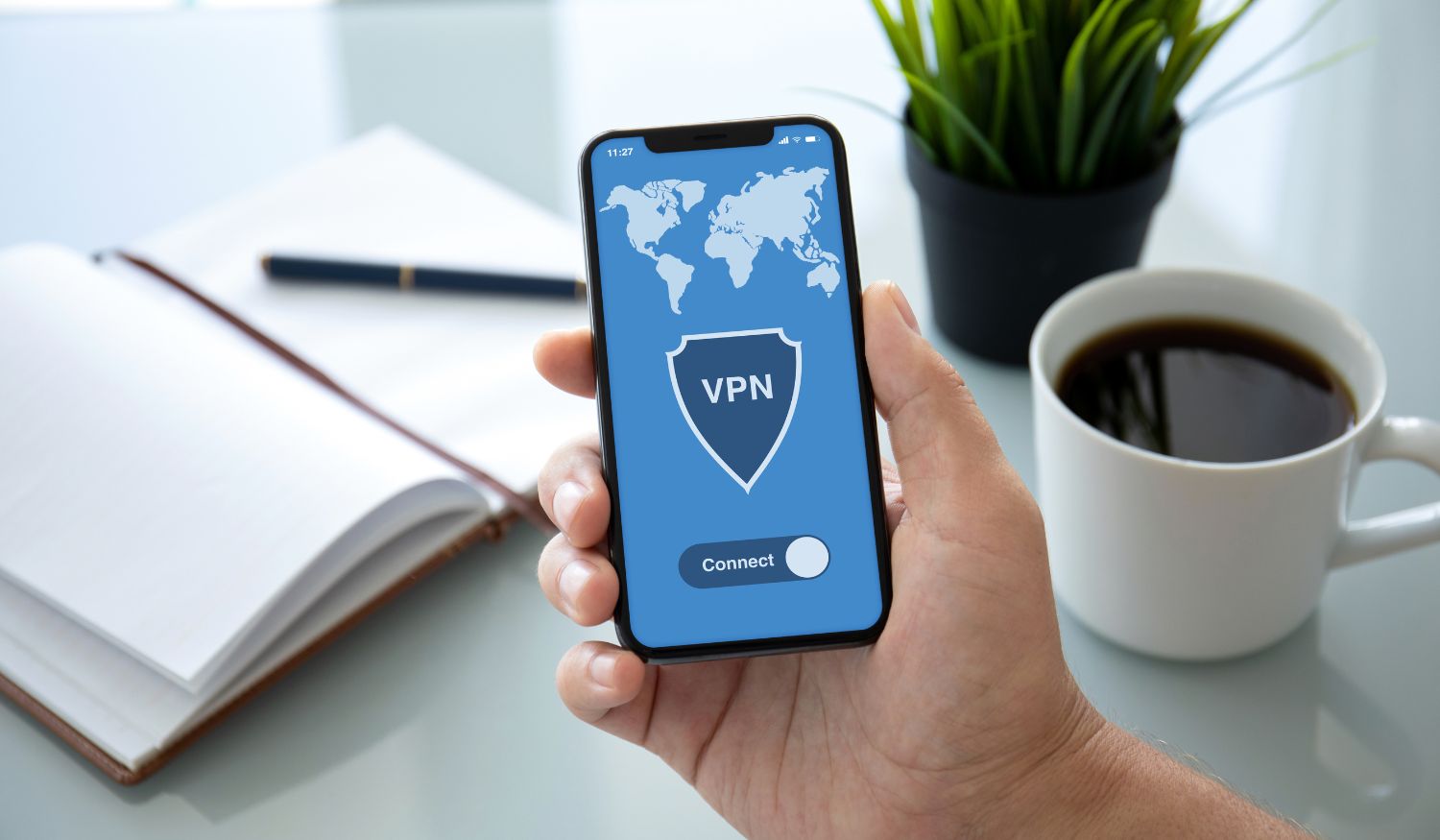