AI4Cybersecurity or Cybersecurity4AI: The Endless Loop
A key characteristic of AI systems is their capability to infer and, therefore, provide predictions, content, recommendations, or decisions from data, which can influence physical and virtual environments. While the benefits of AI4Cybersecurity can be easily identified, with the improvement of threat detection and analysis, attack prediction, forensic analysis, resilience and business continuity, to name just a few, the challenge of protecting AI systems, Cybersecurity4AI, may not be as easily specified. AI systems and models are evolving rapidly and have been embedded into our activities before adequate awareness and literacy regarding its risks and their impact on humans’ fundamental rights, health and safety were clearly stated. How can we guarantee that current cybersecurity solutions and procedures are enough to protect AI? Will we use AI to protect and secure AI? And how to protect the AI that protects AI? We can easily get into an endless loop.
If we care to pay attention, we have various examples and lessons to learn from situations where many ubiquitous technologies are deployed without appropriate cybersecurity and privacy requirements integrated therein. For instance, IoT/IoMT are areas where, if security is at all present, it is commonly at a very basic and non-adequate level. Usually such devices, which closely collect and communicate humans sensitive or environmental data, and integrate with other wireless or wired infrastructures, can promote the cascading and propagation of vulnerabilities throughout a wider surface attack. IT infrastructures integrate both hardware and software, which can originate from different vendors/suppliers, and are usually set within a (somewhat complex) supply chain and a physical infrastructure, where different humans can have different goals, needs and experiences.
Are we following the same path with AI? We cannot. AI systems may quickly evolve to take autonomous decisions and adapt according to their own judgement. Such compounded and intricate ecosystems, if not well grounded in clear, adequate and safe directives, can easily be out of human control and oversight. With their ability to produce novel and potentially impactful outputs, AI systems and models can introduce unique challenges such as: managing the integrity and bias of input and generated content, safeguarding against misuse, unauthorized attempts by third parties to alter its use, behaviour or performance, manage and respond to AI specific vulnerabilities such as data poisoning or adversarial attacks, accidental model leakage, unauthorized releases, circumvention of safety measures, unauthorized access or model theft, and ensuring compliance with evolving regulatory standards and legislation.
So, how can AI systems be cybersecure?
What Ethics and Legislation may have to Offer
The High-Level Expert Group on Artificial Intelligence (AI HLEG) in Europe developed seven non-binding ethical principles for AI which are intended to help ensure that AI is trustworthy and ethically sound. Although an objective description of each principle with some general guidance is provided, no concrete ways, examples, procedures or technologies to achieve them are presented. Those principles are summarized in the following table, based on the content available at https://digital-strategy.ec.europa.eu/en/library/ethics-guidelines-trustworthy-ai:
AI HLEG Ethical Principles |
AI systems developed and used to: |
Human agency and oversight |
serve people, respect human dignity and personal autonomy, and function in ways that can be appropriately controlled and overseen by humans |
Technical robustness and safety |
be resilient against attempts to alter its use or performance or unlawful use by third parties, and minimize unintended harm |
Privacy and data governance |
comply with privacy and data protection rules, while processing data that meets high standards in terms of quality and integrity |
Transparency |
allow appropriate traceability and explainability, while making humans aware that they communicate or interact with an AI system. Duly inform deployers of capabilities and limitations and affected persons about their rights |
Diversity, non-discrimination and fairness |
include diverse actors and promote equal access, gender equality and cultural diversity, while avoiding discriminatory impacts and unfair biases |
Social and environmental well-being |
be sustainable and environmentally friendly in a way to benefit all human beings, while monitoring and assessing the long-term impacts on the individual, society and democracy |
Accountability |
ensure responsibility and accountability for AI systems and their outcomes, before and after their deployment and use |
Similar requirements such as: human oversight, transparency, explainability, accountability, safety, trustworthiness or responsibility have been lately inscribed into current legislation, directives and frameworks all over the world (e.g., EU AI Act, NIST AI Risk Management Framework, the AI and Data Act in Canada or the Artificial Intelligence Law of the People’s Republic of China, and others). However, as legislation usually works, there are no objective procedures and associated technology on how to implement such complex concepts into the practice of digital infrastructures and AI.
Moreover, seemingly different legislation and directives may overlap or do not complement themselves as needed. For example, lately many regulations, directives and legislations have been defined by the EU to regulate the protection of fundamental rights, safety and well-being of the EU citizens, including cybersecurity and data privacy, both in physical and virtual spaces.
However, just as the AI Act itself demonstrates (https://eur-lex.europa.eu/legal-content/EN/TXT/?uri=CELEX%3A32024R1689), different regulations may overlap, and there is the need to provide clarification between their interdependencies, and common aspects of AI systems cybersecurity are merged into the requirements of the various legislations. To give a concrete example, when it comes to biometric systems for surveillance, these need to be compliant with, at least, the (EU) AI Act, the (EU) General Data Protection Regulation (GDPR), the Directive (EU) 2016/680 and the (EU) Cyber Resilience Act. If they are integrated into critical infrastructure domains, the NIS2 Directive must also play a role.
Another example of a legislation dependency, which can seriously affect the way compliance implementation is set, is the fact that the EU AI Act trusts and delegates its privacy and personal data processing requirements to GDPR (https://eur-lex.europa.eu/eli/reg/2016/679/oj/eng). For instance, GDPR Privacy by Design (PbD) principles mostly integrate scattered and general measures that can be picked at each entity’s will, such as minimization, purpose limitation, pseudonymization or accountability, to name a few. However, are these PbD principles enough to integrate into more complex processes as mandated by the AI Act such as, for instance, lifecycle risk and quality management, provision of AI literacy to humans, or keep up with AI models and systems evolution to guarantee that personal data is securely processed during AI models evolution throughout their lifecycle? Training, literacy and awareness can be a successful vehicle for tackling human factor vulnerabilities while promoting the prevention of personal data breaches. However, there is no clear advice on this issue in GDPR and, therefore, no guidance for AI systems in return.
The need for a clear regulatory harmonization with simple and easy to understand language and goals is essential for the correct and uniform application and compliance with those regulations, to assure proper continuous auditing, correction and resilience.
Risk Management: Your Best Friend?
How do you start understanding and putting those essential requirements into practice? One way is to focus on one of the main cybersecurity practices: Risk Management. Risk management integrates risk identification, assessment, addressing, monitoring and reviewing. This practice constitutes the basis to “know thyself”, to identify current vulnerabilities as well as strengths, and to take the most appropriate informed decisions on the best action to go forward. This can guarantee a more accurate and robust cybersecurity strategy. Taking cybersecurity decisions and measures with inaccurate or incomplete data may have a very negative impact on systems and humans’ protection by failing to have an integrated approach to the whole ecosystem. And as we know in security, we need to defend all, as one vulnerability may be enough to take down whole infrastructures.
AI systems can range from unbearable risk to low risk. The former are considered a clear threat to people’s safety, health and fundamental rights and must be banned from use; to ensure traceability and transparency, the latter should, nevertheless, establish appropriate documentation of risk assessment and subsequent management throughout the system’s lifecycle, as AI systems may evolve and integrate unforeseeable risks. Such unforeseeable or evolving risks are the main reason why risk management is crucial. Digital infrastructures are not static in nature. They are dynamic and the risk framework needs to accompany that evolution. When those infrastructures include AI systems or models, such unpredictability and change can evolve at a non-predefined pace and new or unheard-of risks can arise. For this reason, maintaining continuous risk monitoring and assessment is even more relevant.
But is risk management enough to leave the loop between AI and Cybersecurity?
Leaving the Loop
Risk management is a good essential step but certainly not enough in the long run.
We need to start practicing what we have been “preaching” for a long time now: to ideate, develop and deploy AI systems (or in fact any system or technology), with cybersecurity, privacy, ethics and legality by design and by default. Only this way can we build and use resilient and trustworthy systems, always ready to adapt, proact as well as react and fail safe, and with the main goal to protect humans, their fundamental rights and their activities. An AI system and technology needs to be treated as a living (and always changing) organism, which needs to be nurtured, protected and provided with continuous and robust validation, training, testing, monitoring and supervision.
We have had some good practice and technology built over the years to help promote this nurturing. However, in order to leave the loop, we need to complement these with some strategic points:
- Change the research and innovation paradigm: AI that is trusted and secure, needs to integrate trust and security research and innovation from day one to ideate solutions that have all the mentioned requirements by design and by default. Not surprisingly, trust may also come from zero trust architectures and models. Moreover, building resilient systems needs to be thought out and developed both at a more diverse as well as at a personalized level. Which means, build to adapt to the goals, context and humans that will interact or benefit from the system. One-size-fits-all solutions are not the answer anymore and we need to use the advantages and evolution of AI to help build such adapting and dynamic solutions. Research and innovation in cybersecurity for AI must be integrated and customized to provide continuously protected and resilient systems.
- Build robust education and training strategies: even in automated situations using AI we need to promote human oversight and responsibility. Awareness and expertise on this front need to be raised so that humans can also accompany the paradigm change mentioned in the previous point. Training and awareness need to include simulations of “real” human oversight of systems and in understanding, detecting and handling incidents on specific contexts within AI digital ecosystems. Theoretical and general knowledge is still relevant but cannot constitute the main part of training and awareness sessions. Many times, soft skills like analytics, critical thinking, fast decisions, communication and organized methods, are level to level with more technical knowledge and must integrate the main requirements of high-level education and training programs. If we are preparing the systems of the future, we need also to prepare the people that will use, oversight and supervise those systems, much beyond and faster than what is happening today.
- Promote “trusted insiders”: a term I am coining here, to explain how we can promote trustworthiness, responsibility and oversight into AI systems. This includes the integration of agents, both humans and technology, into systems’ functioning. “Trusted insiders” can help the continuous verification and integration of protection and safety into AI design. Technology needs to be built to integrate human oversight, creating a close relation between both (humans and technology), enacted by transparent messages and clear notifications for humans to easily and quickly identify and help find resilient solutions in real-time. This partnership between both “Trusted insiders”, needs to comprise 24/7 vigilance, to monitor, predict, avoid or detect incidents and handling them, as soon as possible. We cannot simply delegate responsibility and trust to the systems themselves. AI will not replace humans but will potentially create diverse and complementary human teams for its continuous supervision and improvement.
- Promote shared responsibility with knowledgeable and appropriate human governance: people and systems do not work and thrive alone. The more we collaborate the more we will be interested in performing better and working for the same goals and needs. Again, this is something we preach all the time, collaboration is key, but the practice is very far from this. Still in such a global world, more connected than ever, we need to have adequate governance and policies to (finally) learn how to communicate and collaborate for the benefit of all. We have great opportunity to do so.
To leave the loop, we need to break current paradigms and engage in improving and securing emerging technologies faster, always with the human in the loop. The stated points can promote interest in protecting and defending people and systems in an integrated and customized way. If the majority is leading this path, then this trend will prevail. People will be better prepared to promote best practice and, together with systems, they will also be prepared to do it by design and by default.
We do not need to start from scratch. We can consider initiatives such as the Global Council for Responsible AI (GCRAI), or similar, which can support AI technology that:
- is ethical, responsible, secure, and aligned with global public interest.
- supports and strengthens the future of AI through education, leadership, certification, and advisory services that are centered in human dignity, innovation, and international cooperation.
- promotes knowledge, training and culturally relevant education, diversity and inclusion of collective wisdom and expertise as well as innovators and visionaries dedicated to building a safer and more responsible future where technology serves humanity.
And that, for me, is what technology should be all about.
Share this
You May Also Like
These Related Stories
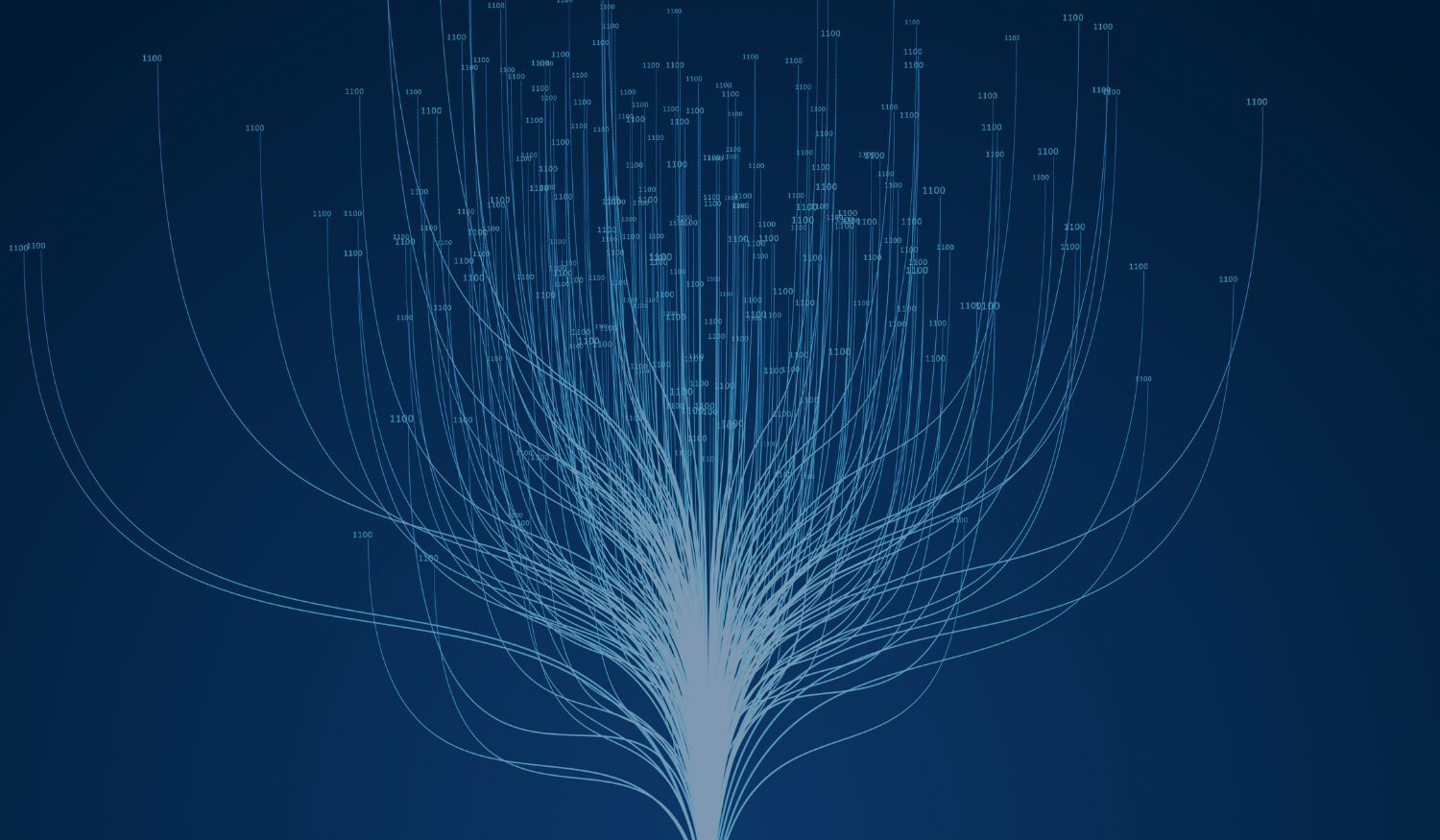
AI & Cyber Law: Taming the Digital Genie
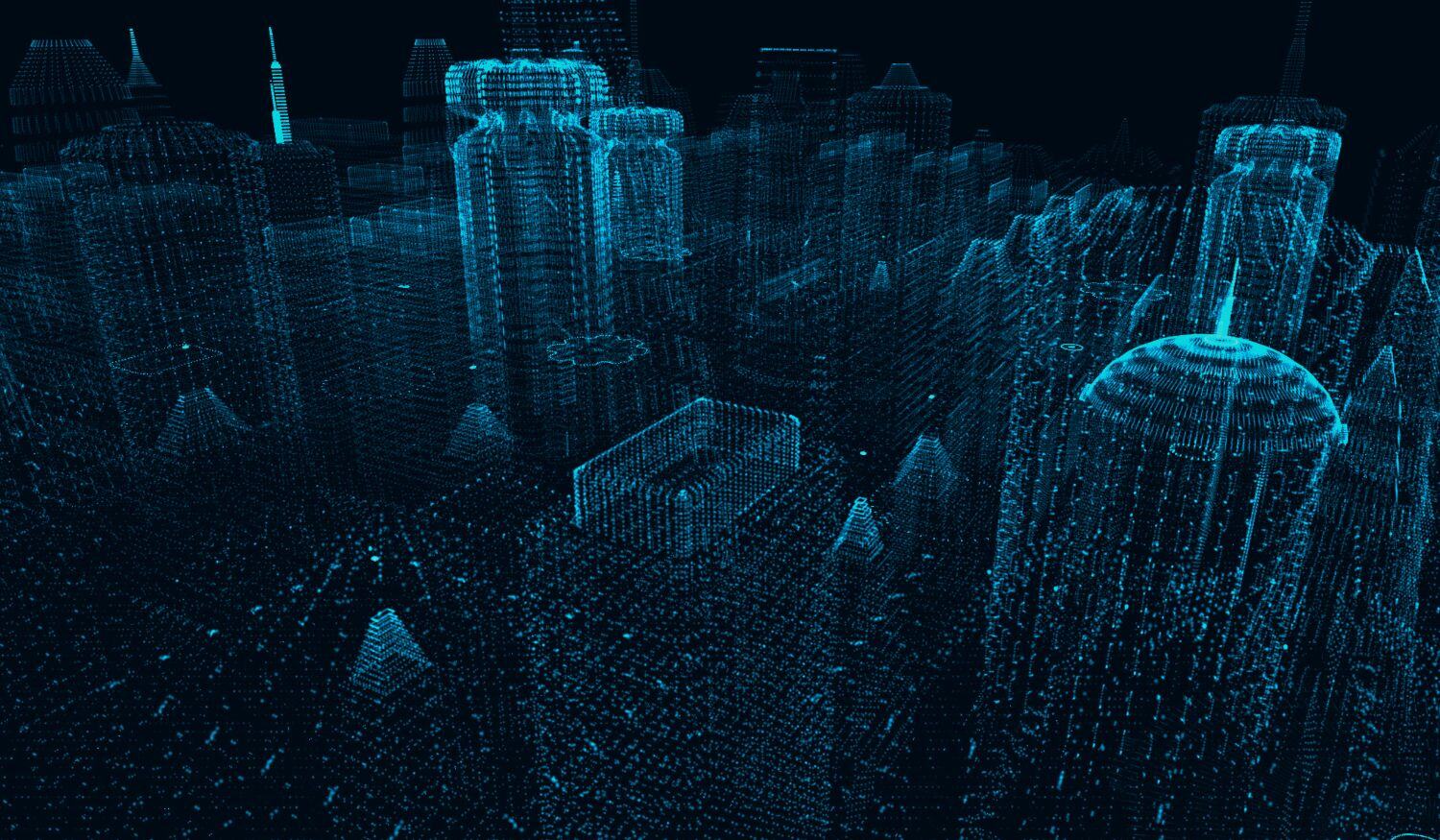
An Introduction Agentic AI in Cybersecurity
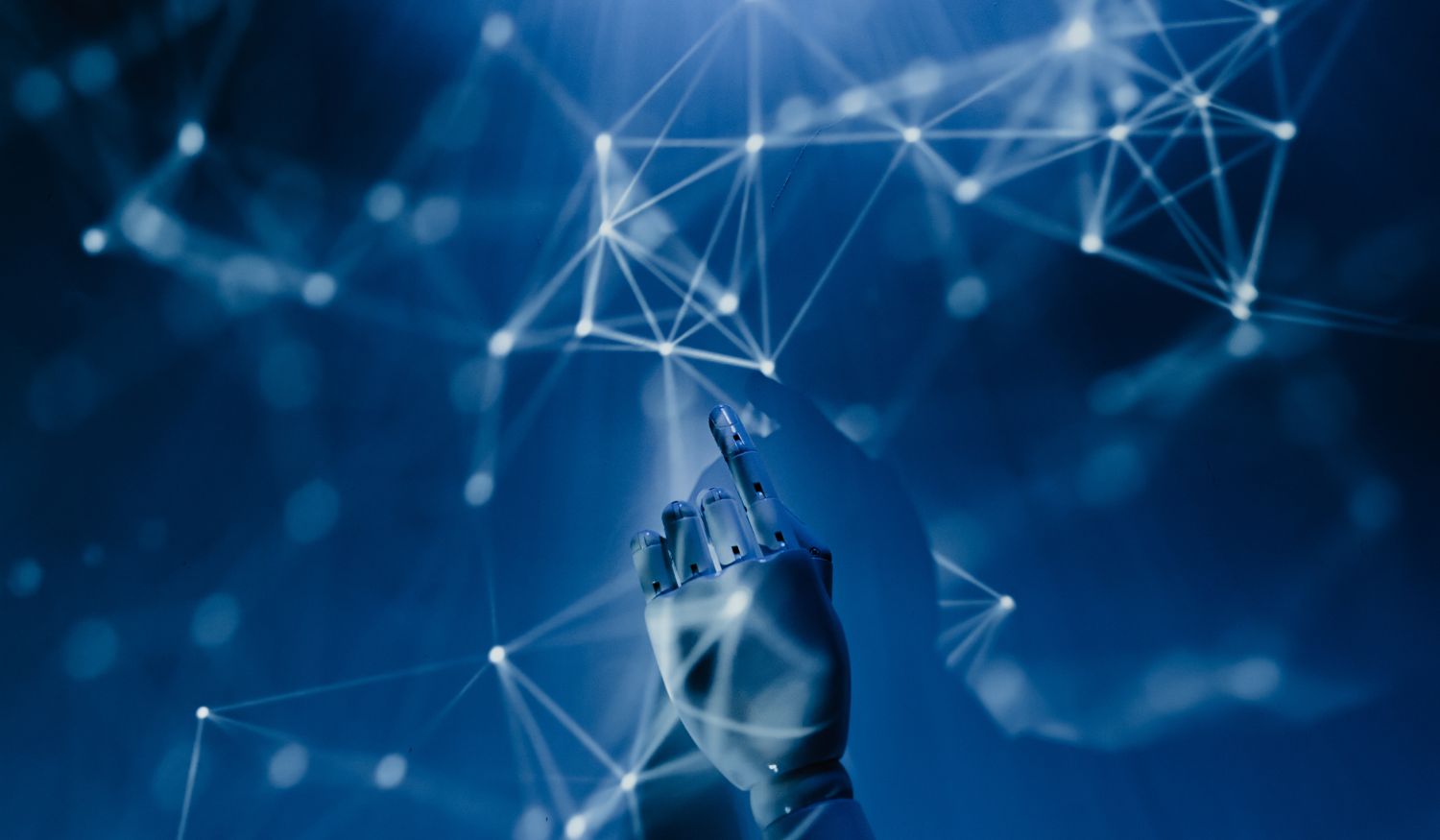