Artificial Intelligence Driven Entropy Model: Revolutionizing Cybersecurity Defense
In an era where cyber threats are becoming increasingly sophisticated, the need for robust defenses has never been more pressing. Traditional cybersecurity measures often prove inadequate against evolving attacks, necessitating innovative approaches. This paper introduces Artificial Intelligence Driven Entropy (AIDE), a ground-breaking concept that combines artificial intelligence with entropy generation to enhance cybersecurity frameworks. By leveraging AI's capabilities to analyze and adapt to dynamic threat landscapes, we can improve the randomness and unpredictability of cryptographic systems. This synergy fortifies data protection and empowers organizations to anticipate and respond to cyber threats more effectively. The need for innovative security solutions is not just a necessity. Still, a call to action and the AIDE concept is a significant step in this direction, revolutionizing how we safeguard our digital environments.
Developing a novel formula for an AI model in cybersecurity that incorporates entropy requires combining information theory, machine learning, and cybersecurity principles. This approach emphasizes the importance of measuring entropy to evaluate and forecast potential cyber threats. By integrating these concepts, we can create a framework that utilizes entropy as a critical metric for identifying vulnerabilities and predicting attacks, ultimately enhancing the effectiveness of cybersecurity measures. This conceptual model is a foundation for further exploration and application in the field, but a definition is needed before we continue. What did I mean by AIDE?
AIDE refers to applying artificial intelligence techniques to enhance the generation and utilization of entropy, which is crucial for creating secure cryptographic keys. By leveraging AI's capacity to analyze vast datasets and identify patterns, this approach improves randomness and unpredictability in security protocols. This innovation strengthens data protection against cyber threats and enables adaptive responses to emerging vulnerabilities, ultimately revolutionizing how organizations safeguard their digital assets in an increasingly complex threat landscape.
What is the major problem? Conventional techniques such as Captcha and Recaptcha are no longer effective in safeguarding data, as advanced AI models can recognize the anticipated patterns and easily circumvent these security measures. As artificial intelligence continues to evolve, it becomes increasingly capable of solving challenges designed to differentiate between human users and automated systems. This shift highlights the need for more robust security solutions that can adapt to the capabilities of AI and provide better protection against potential threats; because of this and the AI's ability to bypass these protections, the exploration of AIDE, which leverages artificial intelligence to enhance randomness and security, addressing vulnerabilities in current cybersecurity frameworks.
The new dichotomy is not only to differentiate between human and machine behavior and encrypted data. Traditional encryption algorithms were not developed considering the incoming of artificial intelligence. Still, AI is here to stay and go, with no denial, and the “bad people” know the weakness of symmetric and asymmetric algorithms as we see the goodness and keep focusing on those. Here is a quick and general review of the most common encryption algorithms (feel free to expand on this topic yourself) and their present risks because of the fast development of AI.
- RSA (Rivest-Shamir-Adleman): This widely used asymmetric encryption algorithm relies on the computational difficulty of factoring large prime numbers for security. However, advancements in AI, particularly with quantum computing capabilities, pose a significant threat as quantum algorithms could efficiently break RSA encryption by factoring these numbers much faster than classical methods. It justifies the topic of this paper.
- AES (Advanced Encryption Standard): While AES is considered robust due to its symmetric key structure and extensive key length options; it is not immune to AI-driven attacks. Quantum computers could utilize Grover’s algorithm to reduce the effective key length, making brute-force attacks more feasible against AES-256, which is currently viewed as secure. It is well known that Grover's algorithm can bypass AES by providing a quadratic speedup in brute-force attacks. Instead of requiring 2 raised to the exponent of the 256 attempts for a 256-bit key, it reduces the effort to approximately 2 raised to the exponent 128 attempts, significantly lowering the security barrier, and this is for now; more is coming.
- DES (Data Encryption Standard): Although largely outdated and replaced by AES, DES remains relevant in discussing encryption vulnerabilities. Due to its relatively short key length (56 bits), AI can accelerate brute-force attacks on DES, making it susceptible to modern computational power and techniques. If you think of a mistake on my end because DES is a 64-bit key, you should think twice before criticizing because out of the 64 bits, eight bits (8) are reserved for parity checks, resulting in an effective key length of 56 bits. Period. DES is equal to 56 effective bits, and not more.
The answer for those asking why 8 bits are reserved is not complex: they verify that the key has not been corrupted, altered during transmission, or processing. The system can detect key errors by enforcing odd parity and maintaining the encryption process's integrity. This mechanism helps prevent potential vulnerabilities that could arise from using an invalid key, ensuring more secure encryption operations, but unfortunately, AI algorithms can “easily” bypass this.
- 3DES (Triple DES): This enhancement over DES applies the algorithm three times to each data block. Yes, we can think about this as increased entropy, but it is insufficient because 3DES still suffers from vulnerabilities similar to DES. AI advancements can exploit its weaknesses, particularly when attackers leverage machine learning to optimize attack strategies.
Now that we better understand current and incoming issues, IADE should be one of many other solutions to secure data and telecommunication processes by combining entropy and artificial intelligence in cybersecurity defenses (essential to talk in plural because as time passes, traditional algorithms became “obsolete” and artificial intelligence still advancing, making the concept of “cybersecurity in layers” more predominant than in any past time).
The essence of this paper is to propose my own AI model that utilizes entropy as a foundational element for enhancing encryption mechanisms in cybersecurity. The main objective is to promote the topic of artificial intelligence-driven entropy (AIDE) and, if possible, listen to your ideas to improve the model.
This model will optimize key generation processes by analyzing data randomness and variability, ensuring more robust cryptographic systems. Integrating machine-learning algorithms will enable the model to adaptively assess entropy levels in real-time, allowing for dynamic adjustment to encryption methods. This innovative approach strengthens data security against emerging threats by leveraging entropy's unpredictability and to be used as an additional cybersecurity control, complimenting between others, the Captcha and Recaptcha models, and based on a prototype that I would like to call “Threat Entropy Index (TEI).”
TEI is an innovative AI-driven model that quantifies the entropy associated with potential cyber threats and will provide a dynamic index that reflects the likelihood of malicious behavior by analyzing data patterns and measuring randomness within network activities. This model will leverage machine-learning algorithms to continuously adapt to evolving threat landscapes, enabling organizations to prioritize defenses based on real-time entropy assessments. Ultimately, TEI aims to enhance proactive cybersecurity strategies by offering a clear metric for evaluating cyber threats' unpredictability and potential risk. Here is the proposed model.
TEI is a conceptual blend of established principles from information theory (like entropy and conditional entropy) applied to cybersecurity. While the individual components (entropy and conditional entropy) are well-known concepts, the specific application as a combined index for assessing cybersecurity threats is a novel approach, even when some other models exist in cybersecurity that incorporate entropy in some form.
In addition, I would like to highlight that this proposal is unrelated to previous investigations related to cybersecurity entropy, mainly the ones that appear to be most relevant, listed here in alphabetical order.
- Detection of Cybersecurity Events Based on Entropy Analysis [Bashurov, V., & Safonov, P. (2023)]
- Entropy Analysis and Ransomware [Vakil, R. (2023, August 18).]
- Higher Entropy Makes the Task for the Attacker Difficult [Maitra, D. (2023, August 14).]
- Why We Need Entropy in Cybersecurity [Redding, M. (2023, March 15).]
The first in the list explores using entropy-based methods for anomaly detection in network traffic. It highlights their effectiveness in identifying cyberattacks by measuring randomness and irregularity in data patterns to enhance cybersecurity measures.
In the second, the author approaches the significance of entropy analysis in combating ransomware, emphasizing its role in assessing the randomness and complexity of ransomware behaviors, thereby aiding organizations in effectively detecting and mitigating potential threats.
The third article emphasizes that higher entropy complicates attackers' efforts by enhancing encryption strength and data unpredictability. This makes it more challenging for cybercriminals to exploit vulnerabilities and successfully breach systems, thereby improving overall cybersecurity resilience.
The last one outlines the critical role of entropy in cybersecurity, particularly in generating secure cryptographic keys. It discusses challenges in obtaining sufficient entropy in modern systems to ensure robust protection against potential cyber threats but does not explore or propose a mathematical model.
I am suggesting a distinct approach that explores how AI can be utilized to improve the generation of entropy, which is critical for secure cryptographic systems. This would enhance overall cybersecurity measures.
References
Bashurov, V., & Safonov, P. (2023). Anomaly detection in network traffic using entropy-based methods: Application to various types of cyberattacks. Issues in Information Systems, 24(4), 82-94. https://doi.org/10.48009/4_iis_2023_107
Maitra, D. (2023, August 14). Higher entropy makes the task for the attacker difficult. Enterprise IT World. https://www.enterpriseitworld.com/higher-entropy-makes-the-task-for-the-attacker-difficult/
Redding, M. (2023, March 15). Why we need entropy in cybersecurity. Quantropi. https://www.quantropi.com/entropy-as-a-service-the-security-boost-of-encryption/
Vakil, R. (2023, August 18). Entropy analysis and ransomware. LinkedIn. https://www.linkedin.com/pulse/entropy-analysis-ransomware-ruhail-vakil
Share this
You May Also Like
These Related Stories
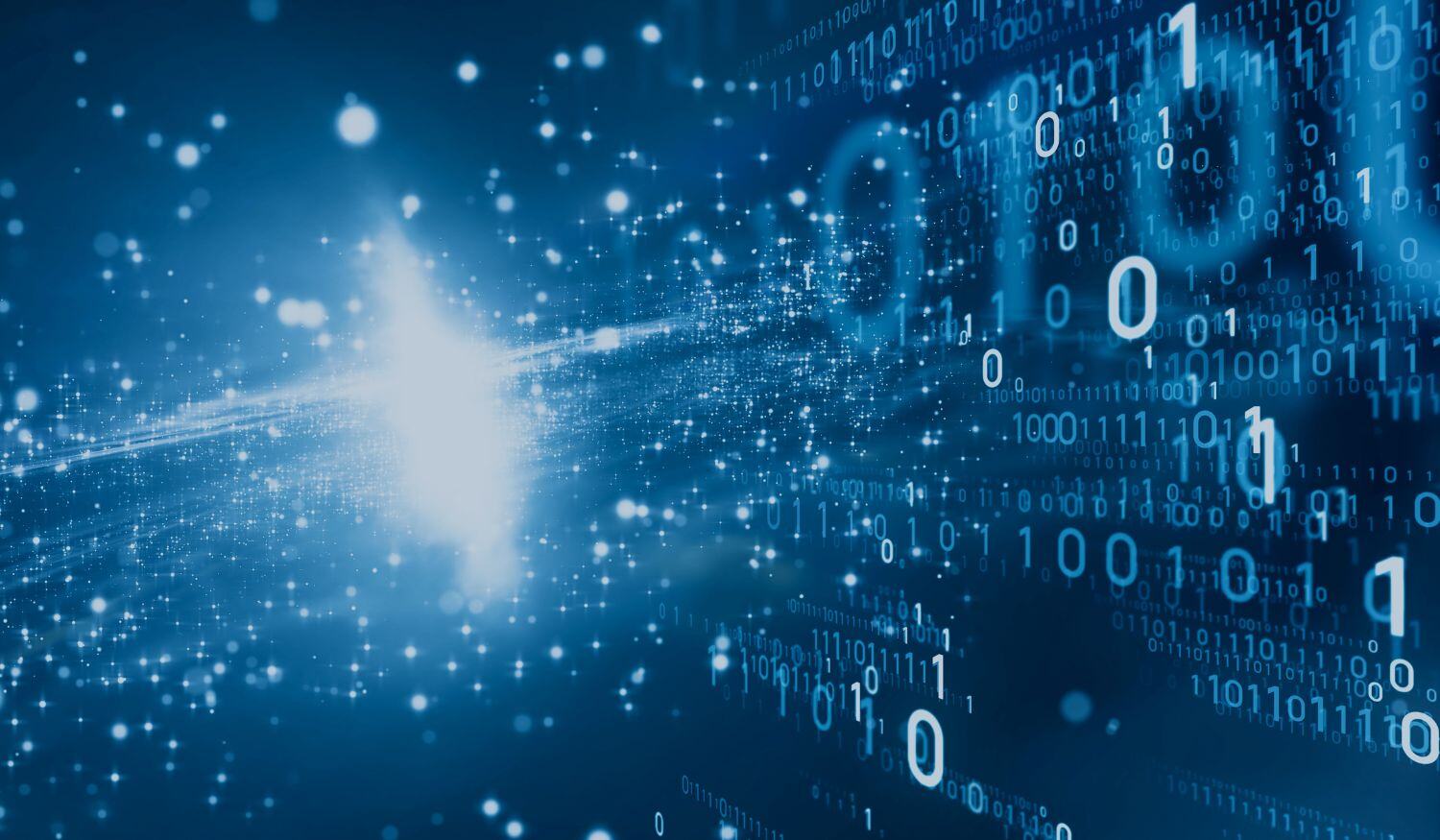
Quantum-Resistant Threat Entropy Index with AI-Driven: Lattice Cryptography
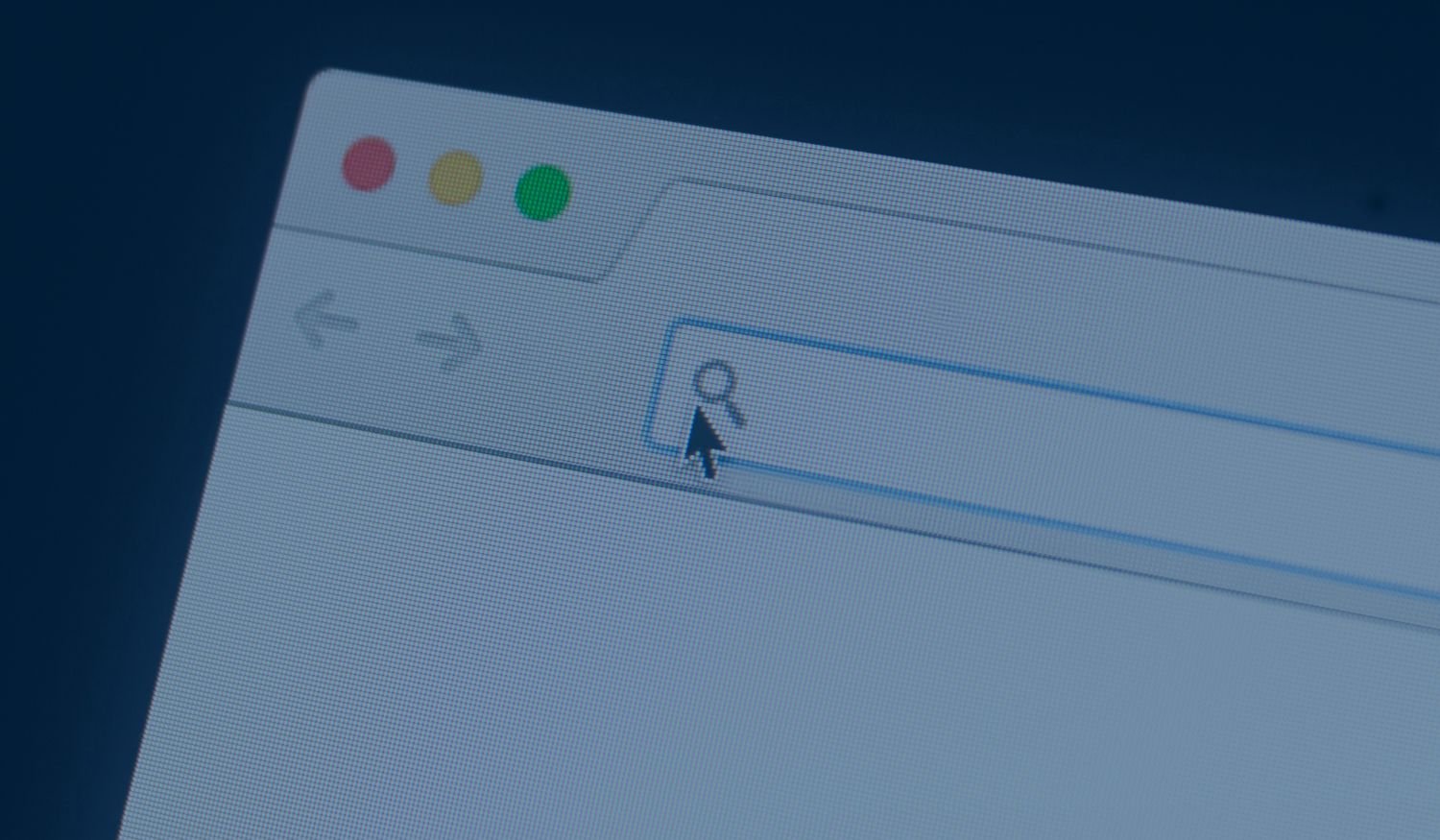
Why You Should Be Using An Enterprise Browser
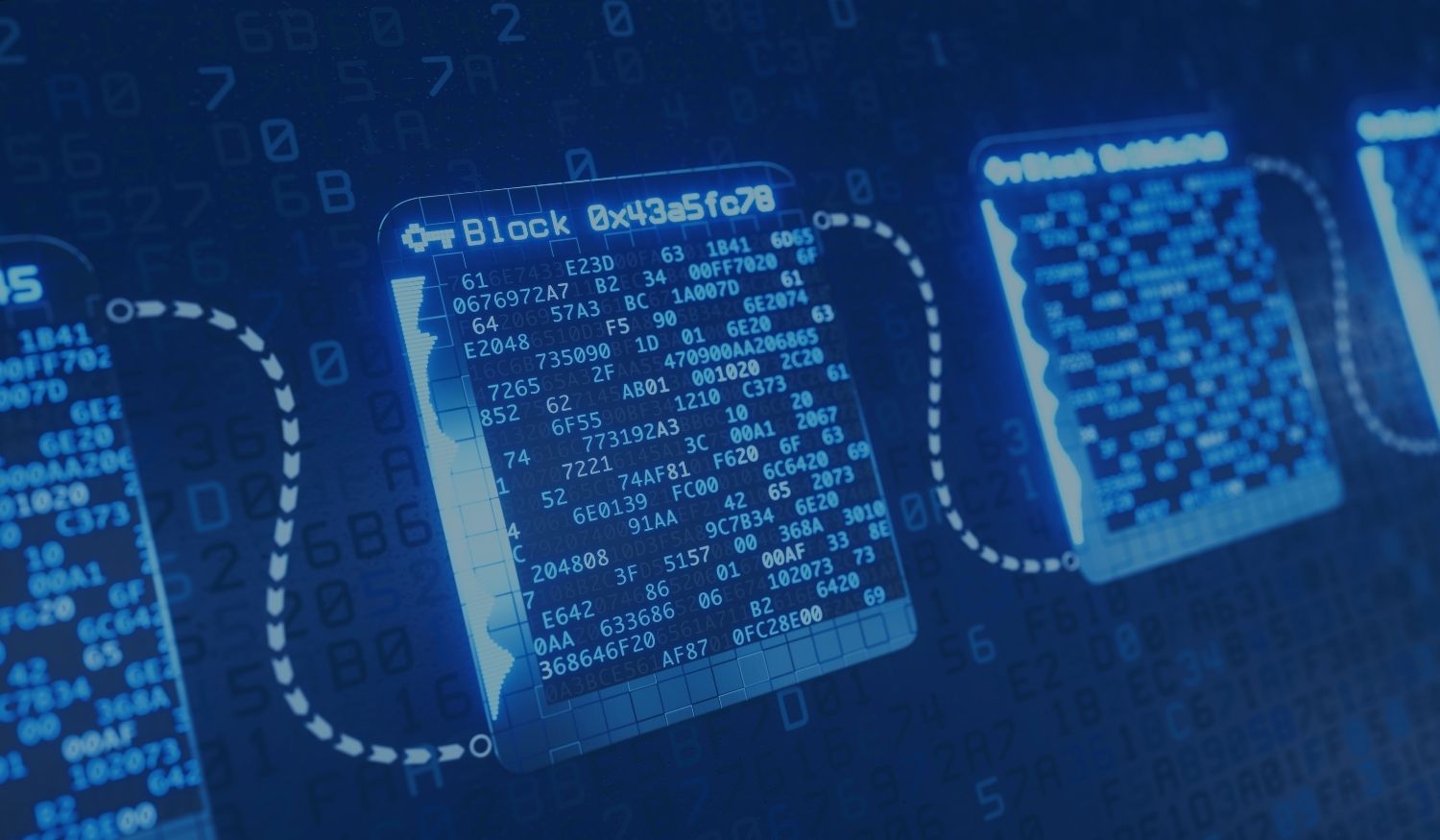