Quantum-Resistant Threat Entropy Index with AI-Driven: Lattice Cryptography
The rapid advancements in quantum computing and artificial intelligence have sparked a new era of cybersecurity challenges, motivating me to explore innovative solutions that anticipate future threats. My previous article, "Artificial Intelligence-Driven Entropy Model (AIDE)", laid the groundwork for understanding how entropy-based AI models can revolutionize threat detection.
This new article explores the concept of a Quantum-Resistant Threat Entropy Index (QR-TEI), integrating AI-driven lattice cryptography to address the looming risks posed by quantum-powered cyberattacks.
Quantum-Resistant Threat Entropy Index (QR-TEI)
Building upon the foundational principles of the Artificial Intelligence Driven Entropy Model (AIDE) and its innovative Threat Entropy Index (TEI), the concept of a Quantum-Resistant Threat Entropy Index (QR-TEI) with AI-driven lattice cryptography represents the next evolutionary leap in cybersecurity.
While AIDE revolutionized threat detection by quantifying entropy to assess malicious behavior, QR-TEI integrates post-quantum cryptographic methods, such as lattice-based encryption, to address the imminent challenges posed by quantum computing. By leveraging AI's ability to dynamically optimize lattice parameters based on real-time entropy assessments, QR-TEI enhances cryptographic resilience against both classical and quantum threats.
This synergy between entropy analysis and quantum-resistant algorithms ensures adaptive defenses evolve with emerging vulnerabilities, positioning QR-TEI as a critical framework for safeguarding digital infrastructures in the post-quantum era.
The Threat Entropy Index (TEI) introduced in my previous article laid the groundwork for leveraging entropy as a critical metric to quantify and predict cyber threats by analyzing randomness within network activities. The proposed Quantum-Resistant Threat Entropy Index (QR-TEI) builds directly on this foundation, addressing the emerging challenges posed by quantum computing.
While TEI focuses on detecting and prioritizing threats based on real-time entropy assessments, QR-TEI extends this concept by integrating post-quantum cryptographic techniques, such as lattice-based encryption, to ensure resilience against quantum-enabled attacks. By combining entropy-driven threat analysis with adaptive quantum-resistant algorithms, QR-TEI not only enhances the predictive capabilities of TEI but also fortifies cryptographic defenses for a future-proof cybersecurity framework.
This evolution from TEI to QR-TEI represents a natural progression in cybersecurity innovation. The dynamic nature of entropy as a measure of unpredictability aligns seamlessly with the requirements of post-quantum cryptography, which demands robust randomness for secure key generation and encryption. QR-TEI leverages AI to dynamically optimize lattice parameters based on entropy fluctuations, creating an adaptive system capable of mitigating both classical and quantum threats. In doing so, it bridges the gap between current threat detection methodologies and the need for quantum-resilient security, ensuring that organizations remain protected in an increasingly complex threat landscape.
Quantum computing introduces profound challenges to the cybersecurity landscape, necessitating innovative solutions that bridge current and future vulnerabilities. My previous publication on the Threat Entropy Index (TEI) established a framework for leveraging entropy as a dynamic metric to detect and prioritize threats in real time.
The proposed Quantum-Resistant Threat Entropy Index (QR-TEI) builds upon this by addressing the emerging challenges posed by quantum computing, which threatens to undermine traditional cryptographic systems. The most pressing concerns include: the ability of quantum computers to crack RSA and ECC encryption via advanced integer factorization algorithms, the risk of "harvest-now, decrypt-later" attacks where adversaries steal encrypted data to decrypt it once quantum technology matures, and the need for truly random number generators (TRNGs) to replace pseudo-random algorithms vulnerable to quantum decryption.
Additionally, quantum computing's scalability raises concerns about mass data breaches and the potential for adversaries to exploit quantum-enabled systems for creating fake digital identities.
To better understand the need of the proposed QR-TEI, it is important to realize that quantum computers have the potential to crack RSA and ECC encryption through advanced integer factorization algorithms, most notably Shor's algorithm. RSA encryption relies on the computational difficulty of factoring large semiprime numbers, a task that is practically infeasible for classical computers due to its exponential complexity.
However, Shor's algorithm, developed in 1994, enables quantum computers to perform this factorization with exponential speed, reducing the time required from thousands of years to mere hours or even minutes, depending on the quantum computer's power.
Similarly, ECC encryption, which uses elliptic curve mathematics for secure key exchange, is vulnerable because quantum systems can efficiently solve discrete logarithm problems that underpin its security. To expand on this topic, my prior article, “Confluence of Lagrange's Theorem and Elliptic Curves,” delves into the mathematical integration of elliptic curves with Lagrange's theorem, highlighting innovative approaches to enhance cryptographic resilience against emerging threats like quantum computing (Noguerol, 2023).
As per the necessity to replace pseudo-random algorithms with TRNGs, it is relevant to understand that pseudo-random algorithms, while effective in many traditional applications, are inherently vulnerable to quantum decryption due to their reliance on deterministic processes that can be reverse-engineered by quantum algorithms like Grover's. To add more, Grover's algorithm enables reverse quantum engineering by efficiently searching for the input x of a function f(x) when given its output y. Unlike classical brute-force methods, which require evaluating all possible inputs, Grover's algorithm uses quantum superposition and interference to amplify the probability of the correct input, reducing the search complexity from O(N) to O (Square Root of N), in where O represents the upper bound of an algorithm's complexity, describing its worst-case scenario. It indicates how the runtime or space requirements of an algorithm grow asymptotically as the input size increases, and N refers to the size of the input or data set being processed by the algorithm. It is a variable used to measure how changes in input size affect the algorithm's performance.
This quadratic speedup allows quantum systems to invert functions or "reverse-engineer" their behavior far more efficiently than classical approaches. By leveraging phase manipulation and diffusion operators, Grover's algorithm systematically narrows down the solution space, making it a powerful tool for tasks like cryptographic key recovery and database searches.
In other words, Shor’s algorithm and Grover's algorithm address distinct challenges in quantum computing, but both have significant implications for cybersecurity. Shor's algorithm is specifically designed to factorize large composite numbers efficiently, leveraging the Quantum Fourier Transform and modular arithmetic to achieve exponential speedup over classical algorithms. This makes it a critical threat to cryptographic systems like RSA and ECC, which rely on the difficulty of integer factorization and discrete logarithms for security.
In contrast, Grover's algorithm offers a quadratic speedup for unstructured search problems, which can be adapted to find cryptographic keys or vulnerabilities in brute-force scenarios. While Shor's algorithm directly undermines public-key encryption by solving its foundational mathematical problems, Grover’s algorithm accelerates attacks on symmetric encryption by reducing the search space for keys. Together, these algorithms highlight the urgent need for quantum-resistant cryptographic solutions to secure data against both targeted factorization and generalized search-based threats.
Wrapping up this section, and to highlight the relevance of the QR-TEI, these algorithms exploit the predictable nature of pseudo-random number generators (PRNGs), allowing quantum systems to efficiently search and uncover the internal states or seeds that drive the randomness.
This vulnerability poses a significant risk to cryptographic protocols, as compromised PRNGs can lead to predictable encryption keys and weakened security systems. To address this, truly random number generators (TRNGs) based on quantum mechanics must replace PRNGs. TRNGs leverage the inherent unpredictability of quantum phenomena, such as photon arrival times or particle decay, ensuring randomness that is immune to quantum attacks.
By integrating TRNGs into cryptographic systems, organizations can achieve the highest level of security against both classical and quantum threats, safeguarding sensitive data in an era of advancing computational power.
QR-TEI addresses these challenges by integrating AI-driven lattice-based cryptography—one of the most promising post-quantum cryptographic methods—into its entropy-driven threat analysis framework. This synergy ensures adaptive defenses capable of mitigating quantum-specific risks while maintaining robust detection mechanisms for classical threats.
By dynamically optimizing lattice parameters based on entropy fluctuations, QR-TEI not only fortifies cryptographic resilience but also anticipates vulnerabilities in emerging technologies like quantum communication networks. This evolution from TEI to QR-TEI reflects a proactive approach to safeguarding digital infrastructures against the unprecedented computational power of quantum systems, ensuring long-term security in a rapidly advancing technological landscape.
The implications of these breakthroughs are profound. With sufficient qubits and error correction capabilities, quantum computers can exploit Shor's algorithm to decrypt RSA-protected data and compromise ECC-based systems, rendering these widely used cryptographic methods obsolete. This threat is exacerbated by the possibility of "harvest-now, decrypt-later" attacks, where adversaries collect encrypted data today to decrypt it once quantum technology matures.
As researchers demonstrate successful factorization of increasingly larger integers using quantum systems like D-Wave's Advantage QPU, the urgency to transition to quantum-resistant cryptographic standards grows, and this is where an “alternative – more secure algorithm” (noted that I used quotation marks), algorithm earn a place to be considered: Lattice’s algorithms (there is more than one).
Lattice-based cryptography operates on fundamentally different mathematical principles compared to Shor's and Grover's algorithms, making it uniquely resistant to quantum attacks. As explained before but to reinforce, Shor's algorithm exploits the structure of number factorization and discrete logarithms to efficiently break RSA and ECC encryption, while Grover's algorithm accelerates brute-force searches by reducing the computational complexity of finding cryptographic keys.
In contrast, lattice-based algorithms derive their security from the inherent hardness of lattice problems, such as the Shortest Vector Problem (SVP) and the Learning with Errors (LWE) problem. These problems involve navigating multidimensional grids of points and are computationally infeasible for both classical and quantum computers, even with advanced algorithms like Shor or Grover.
Unlike RSA or symmetric encryption methods vulnerable to quantum speedups, Lattice-based cryptography ensures robust protection by leveraging problems that remain resistant to quantum computational capabilities, thus securing data in the post-quantum era.
To refresh the definitions, the Shortest Vector Problem (SVP) involves finding the shortest non-zero vector in a lattice under a given norm, typically the Euclidean norm. This problem is computationally hard and forms the basis of many cryptographic systems due to its resistance to efficient solving, even with approximate solutions, while the Learning with Errors (LWE) problem, introduced by Oded Regev in 2005, involves solving noisy linear equations to recover a secret, where the added noise ensures hardness, making it foundational for constructing secure, quantum-resistant cryptographic schemes.
Why was Euclidean mentioned? Simple: because the Euclidean norm reinforces lattice-based cryptography by quantifying vector distances in Euclidean space. These problems resist quantum attacks due to their reliance on geometric complexity in high-dimensional lattices. Post-quantum cryptographic schemes leveraging these norms ensure secure key exchanges and signatures, as they remain computationally infeasible even for quantum algorithms, in a practical manner. Now, going straight to this specific proposal.
To combine the Quantum-Resistant Threat Entropy Index (QR-TEI) with the Threat Entropy Index (TEI), I am proposing a unified framework that integrates entropy-driven threat analysis with post-quantum cryptographic resilience.
The TEI, as developed in the previous model, quantifies the randomness within network activities to detect and prioritize potential cyber threats. This approach can now be enhanced by QR-TEI, which incorporates quantum-resistant cryptographic algorithms, such as lattice-based cryptography, to address the vulnerabilities posed by quantum computing.
The integration ensures that entropy measurements not only detect malicious behaviors but also dynamically inform the selection of cryptographic parameters that are resistant to quantum attacks. This dual-layered system bridges real-time threat detection with proactive cryptographic adaptation, creating a robust defense against both classical and quantum-enabled threats.
Mathematical Framework
Threat Entropy Index (TEI):
More details are on my previous artcile "Artificial Intelligence-Driven Entropy Model (AIDE)".
Integrating this formula into the Quantum-Resistant Threat Entropy Index (QR-TEI) framework (Quantum-Resistant Cryptographic Adaptation)
Introducing a lattice-based cryptographic parameter L(TEI) that adjusts based on the TEI value:
In this case, the symbol used is gamma (γ) commonly used to represent various parameters in mathematics and physics.
The QR-TEI builds directly on my original TEI model by preserving its entropy-based threat detection capabilities while adding a quantum-resistant layer. As entropy levels fluctuate due to network behavior, the system dynamically adjusts lattice-based cryptographic parameters to strengthen defenses against quantum-enabled attacks. This dual-layered approach ensures real-time detection of malicious activity through TEI while proactively fortifying encryption against quantum threats using adaptive lattice algorithms.
By combining real-time entropy-based threat detection with adaptive, quantum-resistant cryptographic techniques, this unified approach ensures that security measures evolve dynamically with the threat landscape.
Enhanced Threat Detection: The TEI component provides a baseline for detecting anomalies and potential security breaches by measuring the unpredictability of network behavior. By calculating the difference between the baseline entropy (H(X)) and the conditional entropy (H(Y|xi)), the TEI model effectively identifies deviations from normal behavior, indicative of potential threats.
Dynamic Cryptographic Adaptation: The QR-TEI introduces a critical layer of quantum resistance through the lattice-based cryptographic parameter L(TEI). This parameter adapts dynamically based on the real-time TEI values, ensuring that as entropy levels rise (indicating increased threat), the cryptographic strength scales proportionally. This dynamic adaptation is crucial for maintaining robust encryption in the face of evolving threats, including those posed by quantum computing.
Quantum Resilience: As quantum computing continues to advance, traditional cryptographic methods become increasingly vulnerable. The QR-TEI addresses this by incorporating lattice-based cryptography [(L(TEI)], which is known for its resistance to quantum attacks. By integrating L(TEI), the framework ensures that cryptographic keys and encryption algorithms remain secure against quantum-enabled decryption attempts.
Balanced Security Approach: The weighting factor gamma (γ) plays a critical role in balancing the importance of entropy-based threat analysis and cryptographic strength. This allows organizations to tailor the system to their specific security needs, prioritizing threat detection or cryptographic resilience based on their risk tolerance and the evolving threat landscape.
Specific Applications of QR-TEI in Cybersecurity
The Quantum-Resistant Threat Entropy Index (QR-TEI) framework offers several specific applications in enhancing cybersecurity as
Adaptive Intrusion Detection Systems (IDS): By integrating QR-TEI into an IDS, the system can dynamically adjust its sensitivity and cryptographic strength based on real-time entropy measurements. For example, when the entropy of network traffic increases, indicating a potential intrusion, the IDS can automatically strengthen its encryption protocols to protect sensitive data from being compromised by either classical or quantum attacks.
Enhanced Key Management: QR-TEI can enhance key management by dynamically adjusting the parameters of lattice-based cryptographic keys based on the entropy of system events. This ensures that keys are regularly updated and strengthened in response to detected threats, minimizing the window of vulnerability and providing robust protection against both current and future cryptographic attacks.
Secure Communication Channels: QR-TEI can be used to create more secure communication channels by integrating entropy-driven cryptographic adaptation into protocols like TLS/SSL. As the entropy of communication patterns changes, the system dynamically adjusts the cryptographic parameters to maintain confidentiality and integrity against both classical and quantum-based eavesdropping attempts.
Dynamic Data Protection: The framework can be applied to data protection strategies, where the strength of encryption adjusts dynamically based on the entropy of data access patterns. This ensures that sensitive data is always protected by the most appropriate level of encryption, mitigating the risk of data breaches even in the event of a successful intrusion or insider threat.
These specific applications leverage the QR-TEI's dynamic cryptographic adaptation to provide a more robust and responsive security posture, ensuring that organizations can effectively defend against evolving cyber threats in the quantum era.
Implementation Considerations for QR-TEI
Implementing the Quantum-Resistant Threat Entropy Index (QR-TEI) requires careful consideration of several factors to ensure its effectiveness and integration into existing cybersecurity infrastructures. Here are some key implementation considerations:
Data Sources: Identify and integrate relevant data sources, such as network traffic logs, system event logs, user activity logs, and security alerts.
Data Preprocessing: Clean and preprocess the data to remove noise, normalize values, and format it into a structured format suitable for entropy calculation.
Entropy Calculation
Baseline Entropy [H(X)] Calculation: Establish a baseline entropy by analyzing normal system behavior patterns. Regularly update this baseline to account for evolving system configurations and normal network activities.
Conditional Entropy [H(Y|xᵢ)] Calculation: Accurately measure the conditional entropy for specific events or anomalies. This requires identifying and categorizing relevant events, such as unusual user behavior or specific attack vectors.
Lattice-Based Cryptography Implementation
Algorithm Selection: Choose appropriate lattice-based cryptographic algorithms (e.g., CRYSTALS-Kyber for key exchange, CRYSTALS-Dilithium for digital signatures) based on performance requirements and security certifications.
Parameter Optimization: Optimize lattice parameters (e.g., lattice dimension, modulus) to balance security strength and computational efficiency.
Dynamic Parameter Adjustment: (please see previous comments on this)
Scaling Factors (α, β, γ): Tune the scaling factors to balance the impact of TEI and lattice-based cryptography on the overall QR-TEI score. This tuning should be adaptive and based on real-world data and feedback. Please note that the adaptability capacity helps a lot.
Real-Time Monitoring: Implement real-time monitoring of QR-TEI values to detect anomalies and trigger appropriate security responses. Set up alerts and automated actions to respond to significant deviations from normal behavior.
Integration with Existing Systems
Compatibility: Ensure compatibility with existing security tools and systems, such as SIEMs, firewalls, and intrusion detection systems.
APIs and Interfaces: Develop well-defined APIs and interfaces to facilitate seamless integration and data exchange between QR-TEI and other components of the security infrastructure.
Performance Optimization
Computational Efficiency: Optimize entropy calculation and lattice-based cryptography to minimize performance overhead. Use efficient algorithms and hardware acceleration where possible.
Scalability: Design the system to scale efficiently with increasing data volumes and network traffic. This may involve distributed processing and parallel computing techniques.
Security and Privacy
Data Protection: Implement robust data protection measures to safeguard sensitive data used in entropy calculation and cryptographic operations.
Access Control: Enforce strict access control policies to limit access to QR-TEI components and data.
Testing and Validation
Regular Testing: Conduct regular testing and validation to ensure the accuracy and effectiveness of the QR-TEI framework.
Simulation and Red Teaming: Use simulation and red teaming exercises to evaluate the system's response to various threat scenarios, including quantum-enabled attacks.
Compliance and Standards
Adherence to Standards: Ensure compliance with relevant cybersecurity standards and regulations.
Documentation: Maintain comprehensive documentation of the QR-TEI implementation, including design specifications, configuration settings, and operational procedures.
By carefully considering these implementation factors, organizations can effectively deploy the QR-TEI framework to enhance their cybersecurity posture and protect against both current and future threats.
The proposed Quantum-Resistant Threat Entropy Index (QR-TEI) builds directly on the foundation of the Threat Entropy Index (TEI) that I developed before by preserving its robust entropy-based threat detection capabilities while introducing a critical layer of quantum-resistant cryptography. The TEI’s ability to quantify unpredictability in system behavior through baseline and conditional entropy provides a precise metric for identifying potential threats.
By integrating this with dynamic lattice-based cryptographic adaptation, the QR-TEI ensures that as threat entropy increases, encryption strength scales proportionally to counter both classical and quantum-enabled attacks. This synergy between TEI and QR-TEI not only enhances real-time threat detection but also future-proofs cybersecurity systems against emerging quantum threats, offering a comprehensive and adaptive defense framework for modern digital infrastructures.
In other words, the QR-TEI framework builds upon the strengths of your original TEI model by adding a dynamic, quantum-resistant layer that enhances overall security posture. This integration ensures real-time threat detection, adaptive cryptographic measures, and robust protection against both conventional and quantum-enabled cyberattacks.
References
Albrecht, M. R., Deo, S., & Postlethwaite, I. (2020). Estimation of algebraic degree for cryptanalysis of multivariate quadratic cryptosystems. Designs, Codes and Cryptography, 88(3), 549-572.
Chen, L., et al. (2016). Report on post-quantum cryptography. National Institute of Standards and Technology.
D’Anvers, J. P., et al. (2018). LAC: Lattice-based compact public key cryptography. IACR Transactions on Cryptographic Hardware and Embedded Systems, 2018(3), 226-257.
Grainger College of Engineering. (2019, October 5). Encryption from Learning with Errors. Retrieved from https://courses.grainger.illinois.edu/cs598dk/fa2019/Files/lecture10.pdf
Harper, T. (2023, January 10). Quantum Computers, Shor's Algorithm, and Post-Quantum Cryptography. Retrieved from https://authortomharper.com/2023/01/10/shors-algorithm-post-quantum-cryptography/
Key Material Blog. (2023, September 1). Learning, but with Errors. Retrieved from https://keymaterial.net/2023/09/01/learning-but-with-errors/
Lyubashevsky, V., Peikert, C., & Regev, O. (2018). Post-quantum cryptography. Proceedings of the IEEE, 106(5), 841-882.
Mosca, M. (2018). Cybersecurity in an era with quantum computers: Will we be ready? Journal of Cybersecurity, 4(1), 69-73.
Peikert, C. (2009, September 1). Public-Key Cryptosystems from the Worst-Case Shortest Vector Problem. Retrieved from https://web.eecs.umich.edu/~cpeikert/pubs/svpcrypto.pdf
Peikert, C. (2016). Lattice cryptography for the internet. In Post-Quantum Cryptography (pp. 197-219). Springer, Cham.
Quantum Computing Stack Exchange contributors. (n.d.). Quantum states are unit vectors... with respect to which norm? Retrieved from https://quantumcomputing.stackexchange.com/questions/2729/quantum-states-are-unit-vectors-with-respect-to-which-norm
Quantum Computing Stack Exchange. (2023, May 7). A quantum k-nearest neighbors algorithm based on the Euclidean norm. Retrieved from https://arxiv.org/pdf/2305.04287.pdf
Noguerol, L. (2024)). Artificial intelligence-driven entropy model aide. Cybersecurity Tribe. Retrieved from https://www.cybersecuritytribe.com/articles/artificial-intelligence-driven-entropy-model-aide
Rubinstein, A., & Guo, N. (2019, May 15). Lecture 14: Learning with Errors. Retrieved from https://web.stanford.edu/class/cs354/scribe/lecture14.pdf
Wikipedia contributors. (2024, April 21). Lattice problem. Retrieved from https://en.wikipedia.org/wiki/Lattice_problem
Note: APA style conventions were not strictly adhered to in this article, as the primary focus is on delivering technical recommendations and insights rather than formal citation formatting.
©2025, Luis O. Noguerol, Advanced Division of Informatics & Technology, Inc. All Rights Reserved. This content is exclusively owned by the author and protected by copyright law.
Share this
You May Also Like
These Related Stories
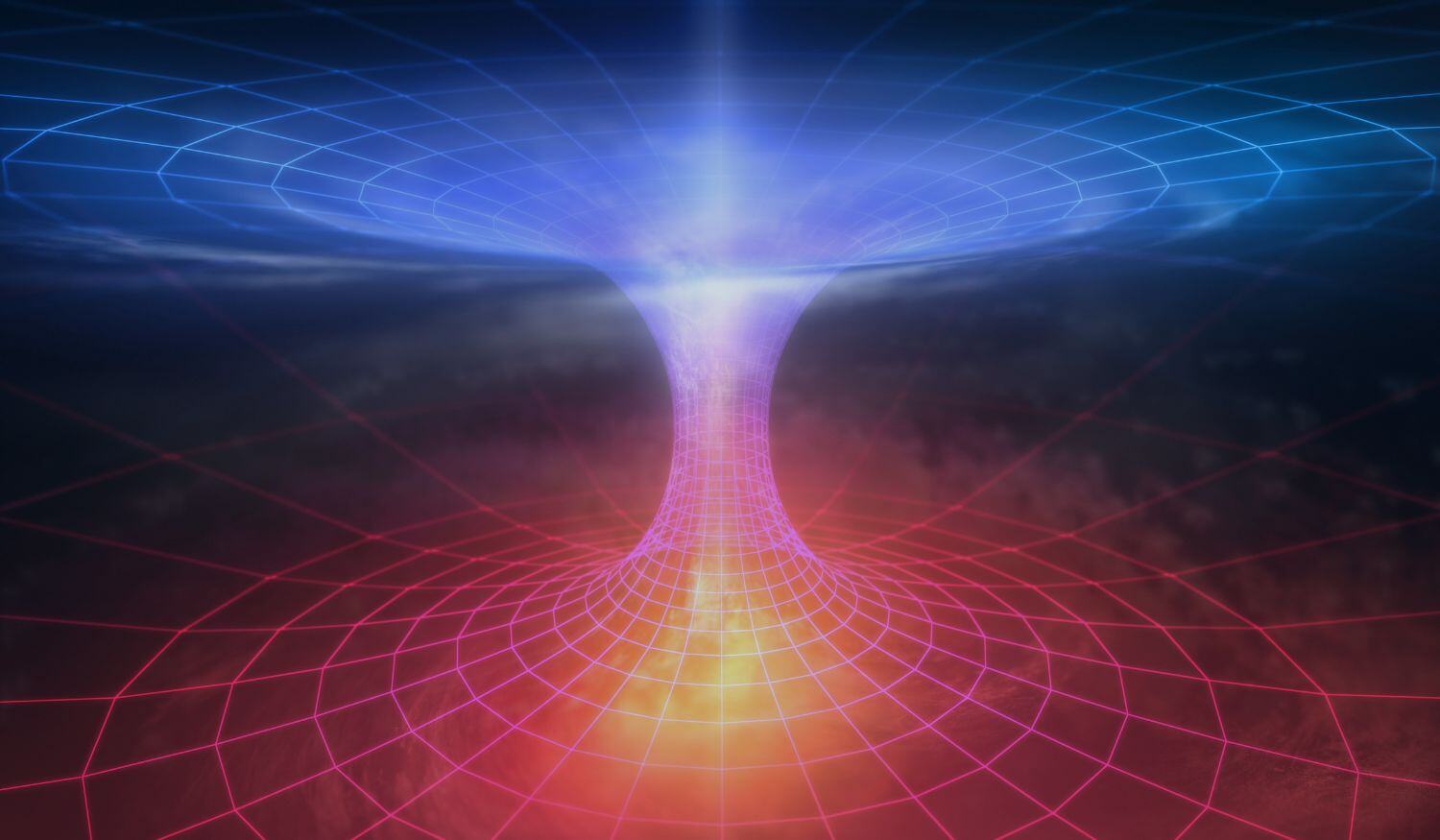
Quantum Y2K Problem Is Huge!
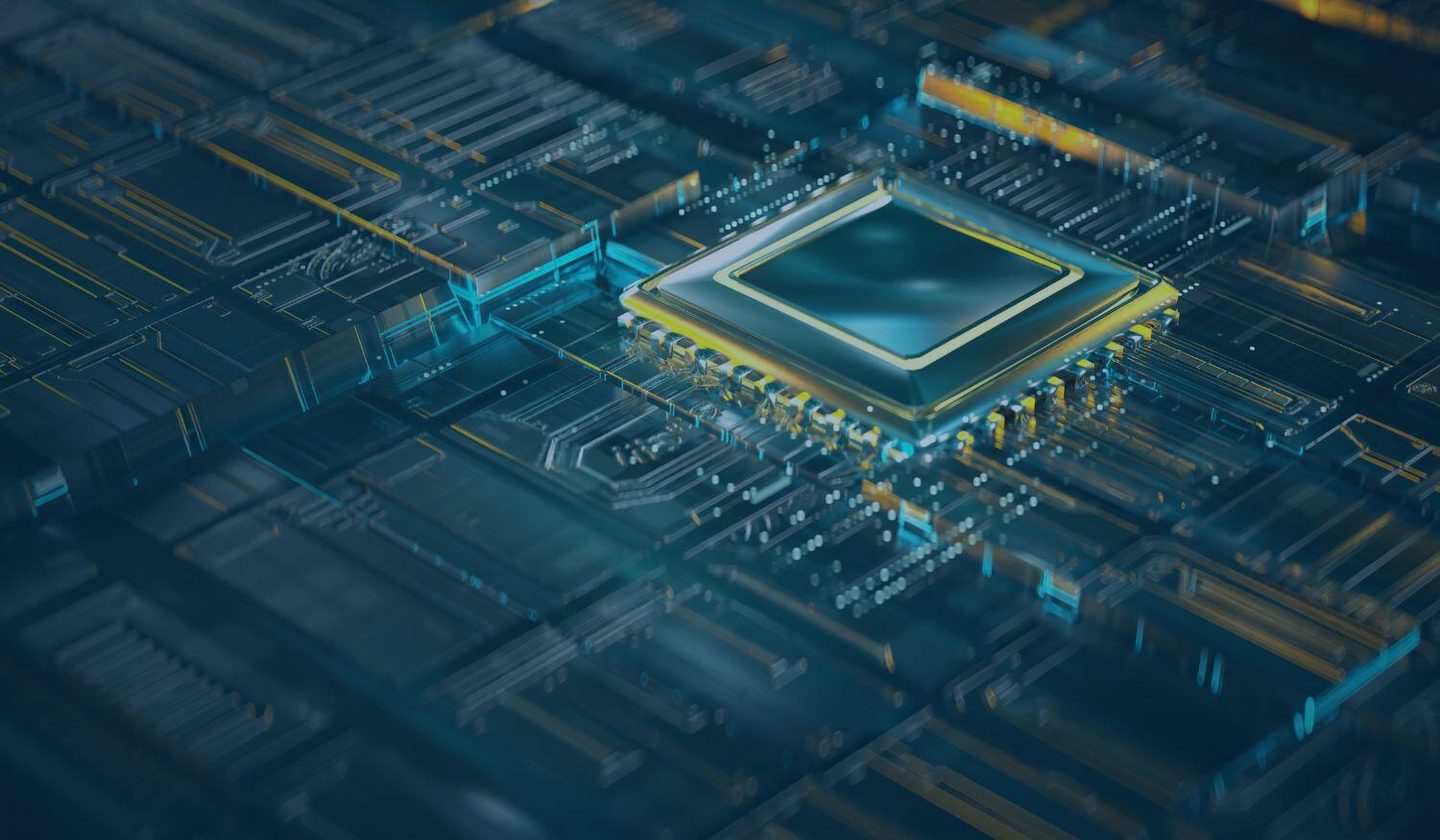
The Race to Decrypt the Past
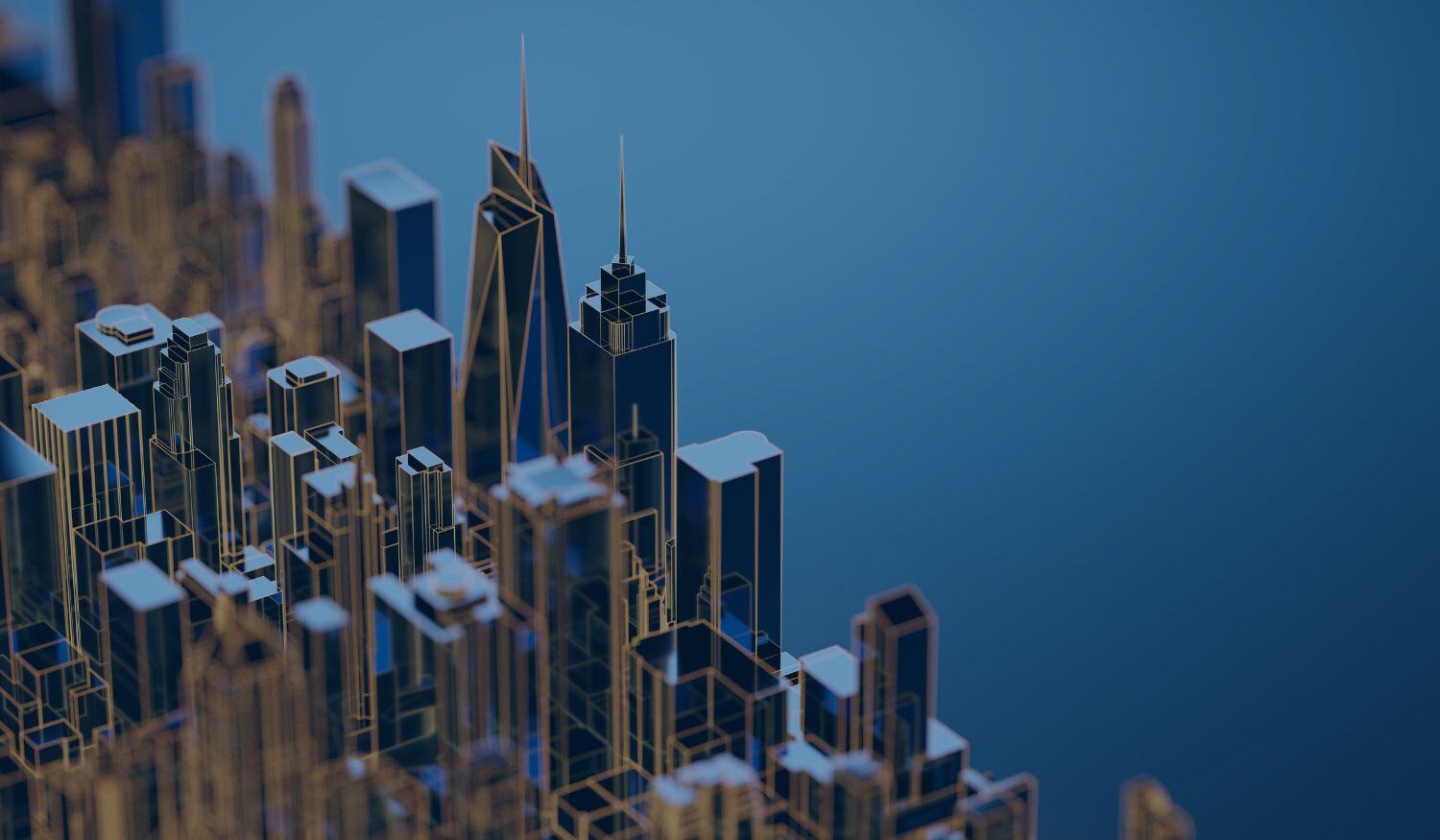