You can listen to this article in audio format by clicking play above
A Call to Action for Security and Data Leaders
We are officially in the era of artificial intelligence, and data has finally been acknowledged ubiquitously as the lifeblood of innovation. As AI technologies like Large Language Models (LLMs) and generative AI continue to evolve, businesses are unlocking unprecedented opportunities for growth and efficiency. However, with these advancements comes a pressing need for data security and governance frameworks.
Take, for instance, a global healthcare organization that implemented AI-driven diagnostics to improve patient outcomes. Their AI models provided highly accurate diagnoses and treatment recommendations by leveraging vast patient data. Yet, this success also highlighted significant risks. The sensitive nature of health data and the AI's need for extensive data access created vulnerabilities such as unauthorized data access and inadvertent exposure of patient information, which could have led to data breaches and severe regulatory non-compliance if not correctly managed.
Consider another scenario involving a leading financial institution that integrated AI to enhance fraud detection. The institution's AI system accurately identified fraudulent activities using proprietary transaction data. However, this implementation also exposed critical vulnerabilities. The AI model's continuous access to sensitive financial information increased the risk of data leaks and unauthorized access, presenting a formidable challenge to the institution's data governance framework.
A last example is a tech company developing an internal AI application to optimize its supply chain management. The company aggregated diverse datasets from departments, including procurement, logistics, and inventory management, to train the AI. While this approach promised to optimize and evolve their operations, it also introduced new risks. Aggregating training data from multiple sources exposed sensitive business information and heightened the complexity of ensuring data integrity and security across the organization.
The Benefits and Risks of AI Models Using Internal Data
Moreover, with its unique blend of specific information, proprietary data offers businesses a chance to sharpen their competitive edge. By refining established AI models using this internal data, companies can achieve unmatched accuracy specially tailored for their specialized tasks. In essence, this means products, solutions, or services that are more attuned to specific customer bases, more responsive to niche markets, and better poised to address unique challenges. However, a new vulnerability creeps in as the AI’s specificity is enhanced. Proprietary data is often the intellectual backbone of a company. When such data feeds into AI models for refinement, it becomes exposed to potential breaches. This isn’t just about data theft; there’s a risk of the AI inadvertently revealing insights about the proprietary data it was trained on, leading to inadvertent data disclosures or even competitive intelligence leaks.
Unfortunately, these scenarios are not unique, and today’s AI is dynamic, not static. Advanced AI frameworks interact seamlessly with data reservoirs, especially in real-time applications like chatbots, predictive analytics, or dynamic pricing models. They extract, process, and act on information in real time. This dynamic interplay elevates user experience, fosters immediacy in decision-making, and ensures that AI outputs are based on the most recent and relevant data available. However, every real-time interaction is a potential point of vulnerability. As AI continuously taps into proprietary data reservoirs, it poses challenges in tracking and securing each data access point. The dynamic nature also means that traditional, static security measures must be improved. Every real-time access and extraction increases the surface area for potential attacks or data breaches.
With the advent of Large Language Models (LLMs) like GPT-4, there’s a realization that while generic models are powerful, there’s unparalleled value in bespoke AI. Large corporations now understand the advantage of tailor-made AI models designed and calibrated precisely for their unique datasets. These enterprise-specific LLMs can offer insights, predictions, and solutions that are laser-focused on specific organizational goals and challenges. However, custom development also means a deeper immersion of AI within the company’s data landscape. When trained on vast swaths of organizational data, these LLMs can unintentionally “memorize” or imprint sensitive information. Moreover, ensuring consistent security and ethical standards is challenging with bespoke models. Each model’s unique nature might necessitate individualized risk assessments, increasing the complexity of AI governance.
While these trends underline AI's evolving nature, they also underscore a significant challenge: data management. The convergence of proprietary data usage, real-time data interactions, and bespoke LLM development has amplified the urgency to address data security. This doesn’t merely pose theoretical questions but translates into authentic and immediate business challenges. At the core of these challenges is data access and management.
Data Governance and Security Strategies
Security and data leaders must prioritize comprehensive data governance and security strategies to navigate these challenges. This involves:
- Proactive Risk Management: Implementing continuous monitoring and real-time risk assessment tools to detect and mitigate potential data vulnerabilities during the AI training and deployment phases.
- Pre-training Assessment: Before training commences, datasets should undergo a rigorous vulnerability assessment. Tools can scan for potential weak points or unwanted data snippets.
- Continuous Monitoring: Even during the training phase, real-time monitors should oversee data interactions and catch anomalies on the fly.
- Data Access Control: Establishing differential access protocols ensures that only authorized personnel and AI models can access sensitive data, thereby minimizing the risk of data leaks.
- Data Classification: Leveraging tools that can automatically classify data based on sensitivity. For instance, it is used to mark data that contains Personally Identifiable Information (PII) or trade secrets.
- Differential Access: Access hierarchies are defined based on the sensitivity classification. Not every developer or AI model requires access to all tiers of data.
- Transparency and Accountability: Maintaining detailed audit trails and visibility panels to track data interactions and ensure accountability across the organization.
- Audit Trails: Logging every access request and modification ensures accountability and provides an invaluable resource in case of a security event.
- Regulatory Compliance: Staying ahead of evolving regulatory landscapes by integrating flexible data security strategies that can adapt to new laws and guidelines. This includes ensuring transparency in AI decision-making processes and upholding ethical standards.
- Education and Training: Continuously educating staff on best data practices to prevent the proliferation of "shadow data" and ensure a culture of security awareness.
Organizations must build resilient frameworks to protect their data assets as we face AI-driven innovation and data security challenges. The future of AI holds immense promise, but it must be pursued with a steadfast commitment to data integrity and security.
By addressing these concerns head-on, we can ensure that AI's potential is realized safely and responsibly, paving the way for a future where innovation and security coexist seamlessly.
Share this
You May Also Like
These Related Stories
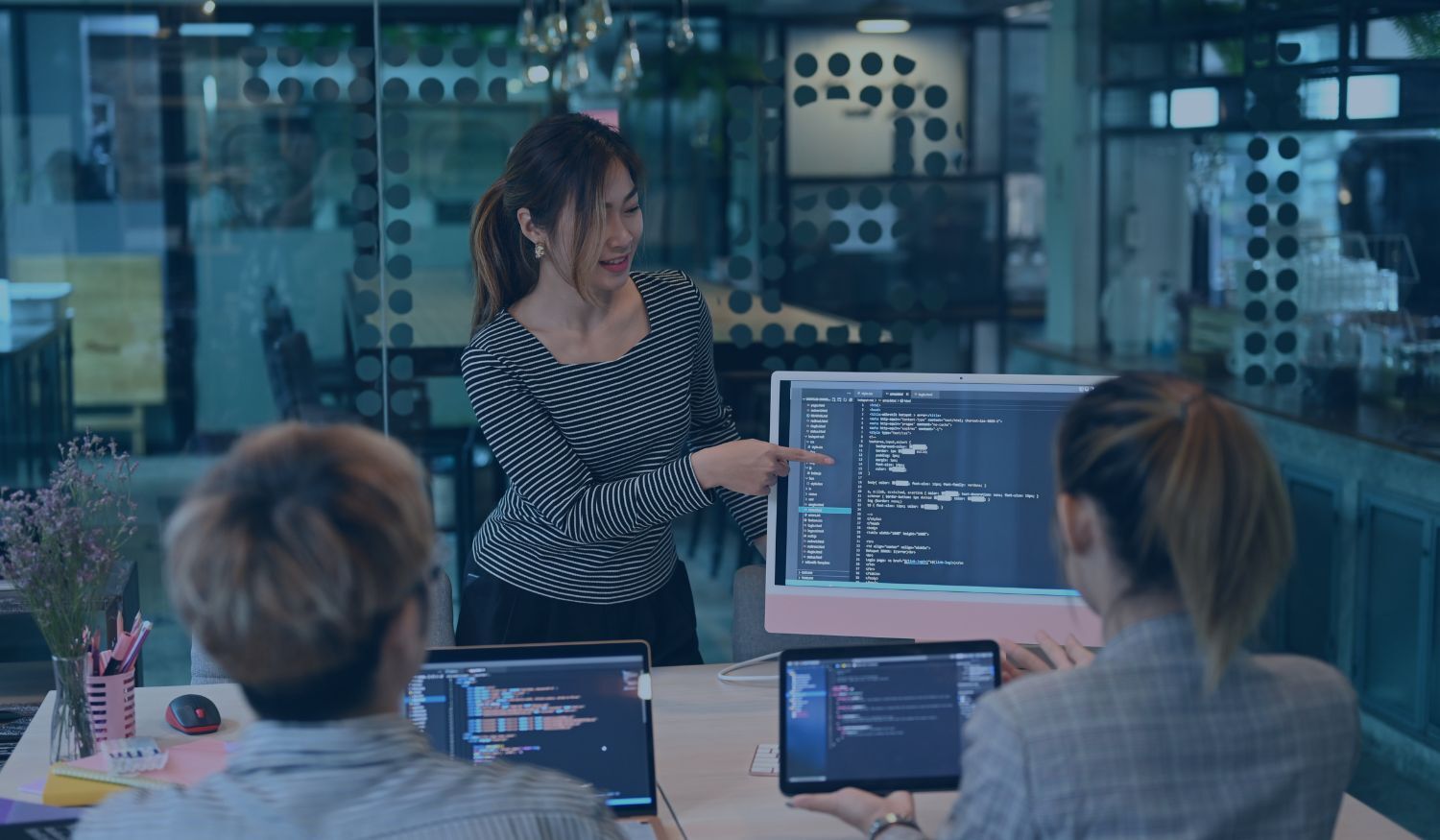
The AI Frontier: Balancing Innovation and Risk in the Enterprise
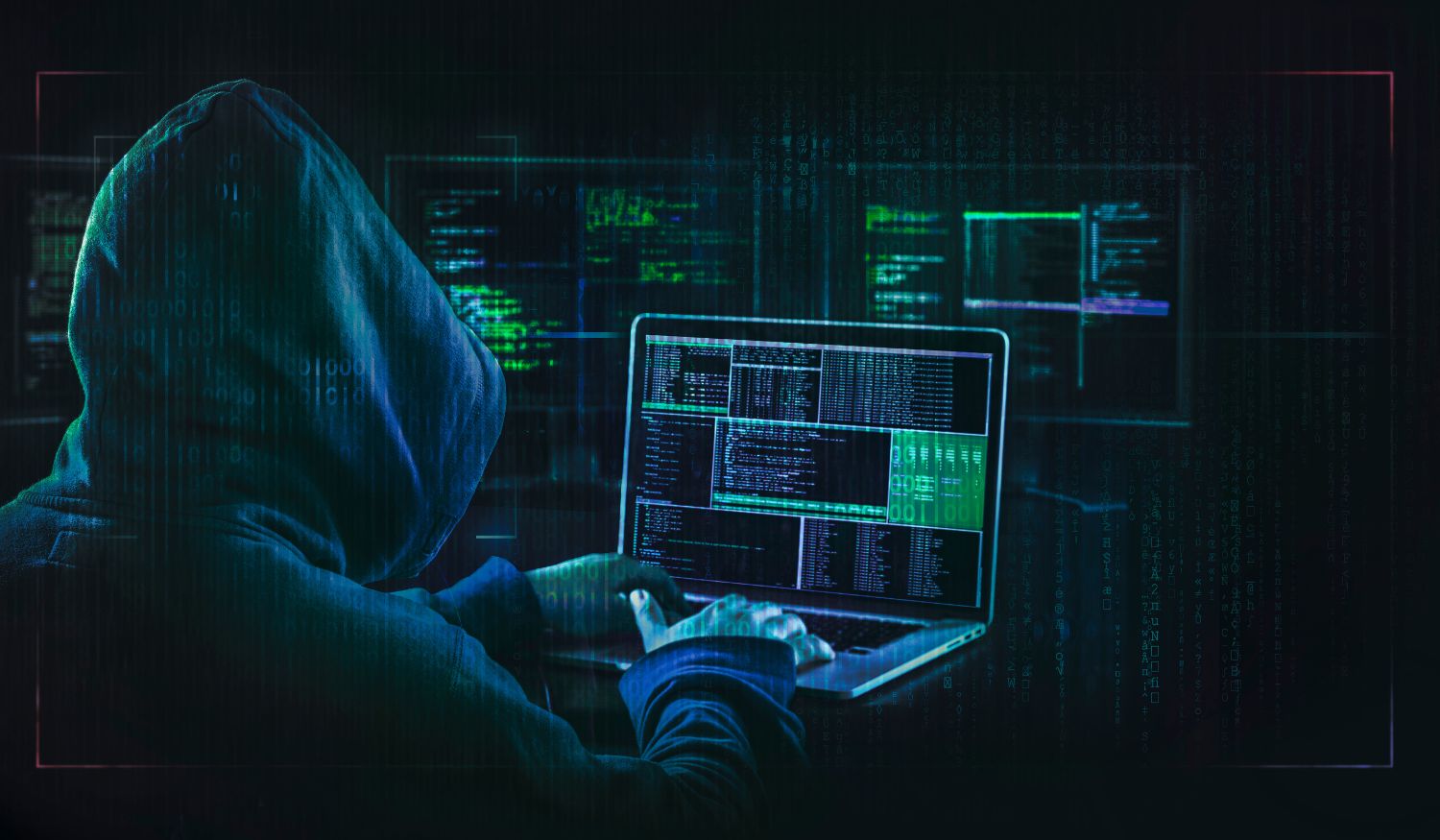
The Dark Side of AI: Unmasking the Malicious LLMs Fueling Cybercrime
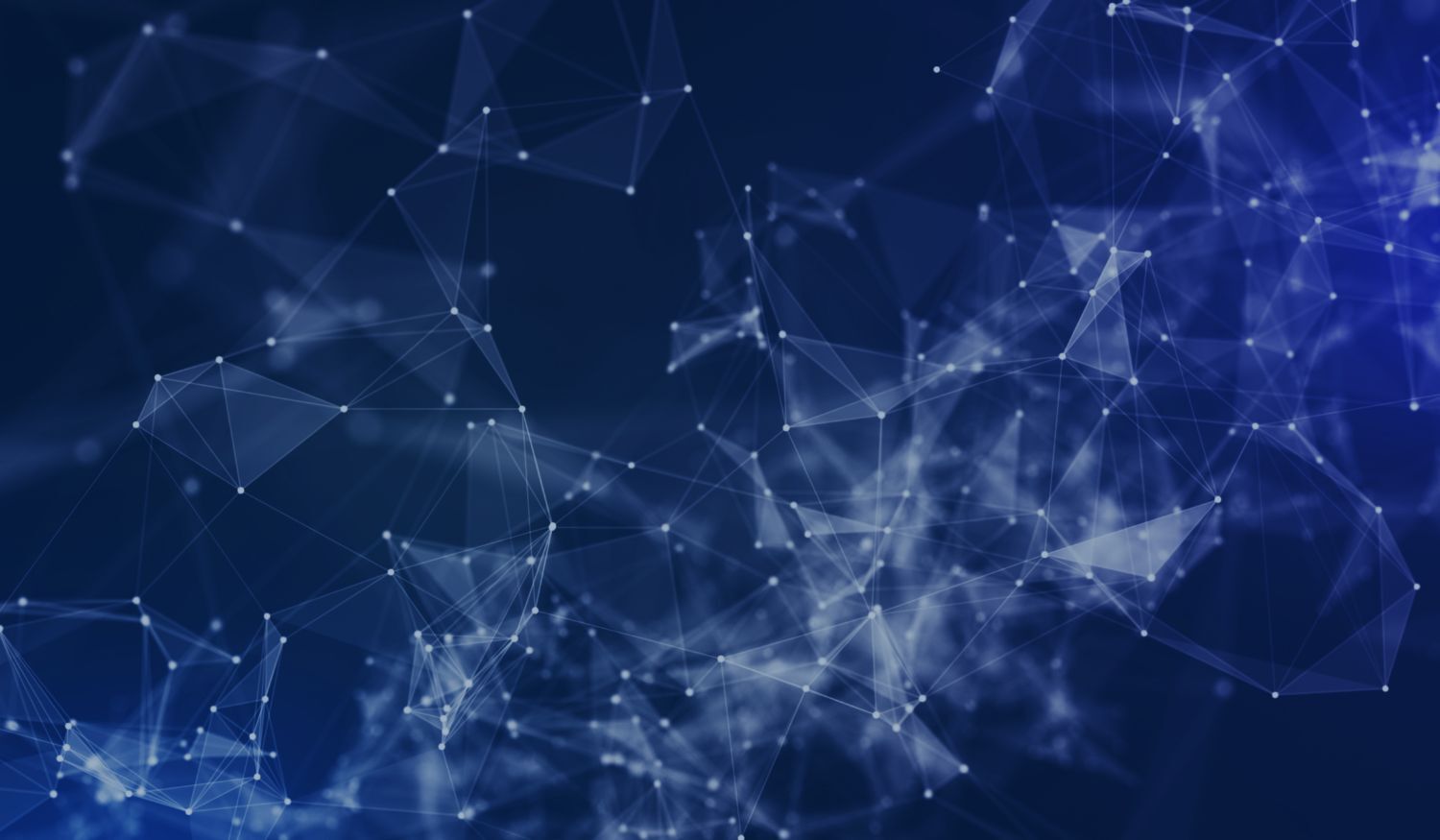