How Cybersecurity Strengthens AI Governance
As Artificial intelligence (AI) systems become more embedded in digital infrastructure, the need for robust cybersecurity measures to ensure their trustworthiness becomes paramount. This article explores the intersection of AI governance and cybersecurity, demonstrating the critical role that CISOs play during the development of AI governance frameworks and the deployment of an AI system in an organization.
What is AI Governance?
AI governance refers to the processes, norms, and technical safeguards designed to ensure that AI development and deployment are safe, beneficial, and human rights compliant. Considering that AI is increasingly integrated into various domains, organizations are prioritizing robust AI governance structures that not only mitigate risks such as privacy infringement, bias, and misuse, but also foster innovation and public trust.
For this goal to be achieved, the following key elements of AI Governance must be considered:
- Ethical Considerations: Ensuring that AI systems adhere to ethical principles, such as fairness, transparency, do no harm, explainability, and accountability.
- Regulatory Compliance: Aligning AI development and deployment with relevant laws and regulations.
- Risk Management: Identifying and mitigating potential risks associated with AI technologies.
- Stakeholder Engagement: Involving diverse stakeholders in the governance process to ensure that AI systems meet organizational needs and values.
A human-centred approach to AI governance necessarily requires input from a diverse range of stakeholders, including technical experts such as scientists and engineers, as well as operational managers, legal and human resources teams, UX designers, and the Chief Information Security Officer (CISO). The integration of these varied perspectives, ensure that the AI systems deployed within the organisation are effective, ethical, and secured.
Trust in AI Implementation
The implementation of AI systems in any organization represents a significant strategic shift that goes far beyond mere technological adoption. At its core, successful AI integration hinges on trust. When AI systems make consistent, fair, and explainable predictions or decisions, stakeholders develop trust in their capabilities.
Trust directly influences whether and how AI systems are adopted within an organization. Low trust environments typically lead to resistance to AI implementation, underutilization of AI capabilities, circumvention of AI systems, and reluctance to share data necessary for AI functioning. Conversely, high trust environments enable full realization of AI's potential through enthusiastic adoption and proper utilization.
Several organizational approaches can enhance trust in AI systems. For instance, developing and/or deploying models that provide understandable explanations for their outputs, testing across diverse scenarios, including edge cases, and continuous monitoring, detecting and addressing performance degradation or data drift. Incorporating robust cybersecurity measures is crucial in this process, as they play a pivotal role in building and maintaining trust in AI systems.
Cybersecurity: Cornerstone of Digital Trust
Within AI systems, cybersecurity extends beyond traditional IT security concerns to encompass the protection of algorithms, training data, and decision-making processes. Unlike conventional threats, AI-specific attacks such as data poisoning, evasion or model extraction are designed to exploit the unique vulnerabilities of machine learning models since they exploit the statistical learning processes that are central to how AI systems work.
As AI continues to integrate into critical sectors like healthcare, finance, and national security, ensuring robust defences against these emerging threats is essential for maintaining trust, reliability, and ethical integrity in AI-driven systems. Digital trust is built on the promise that data and systems are secure and reliable. A breach or compromise undermines immediate operations but more impactfully, can erode stakeholder’s trust in subsequent AI endeavours.
Trusted AI systems must be built upon strong cybersecurity measures. Robust cybersecurity practices serve as the first line of defence against the tampering, manipulation, or exploitation of AI components. Without comprehensive cybersecurity strategies, any AI governance framework would lose its effectiveness. The integrity and reliability of an AI system can only be ensured if all potential points of vulnerability are identified and mitigated.
Zhang et al. (2022) argues that governance policies must mandate cybersecurity protocols throughout the AI lifecycle from data collection and model training to system deployment and monitoring. This analysis reveals that when cybersecurity is integrated from the initial stages of AI development, potential threats can be more effectively anticipated and neutralized.
Many organizations assume that ethical guidelines and compliance measures alone suffice to secure AI systems. However, ethical governance without cybersecurity mechanisms is akin to constructing a high-rise on an unstable foundation. The integration of cybersecurity within governance frameworks is crucial; for example, establishing secure access controls, encryption protocols, and continuous monitoring can significantly reduce the risk of adversarial interference.
Think of "social license" as public permission or acceptance. The same way a restaurant needs customers to trust their food safety standards to stay in business, AI technologies need digital trust to be widely adopted and accepted in an organization, and more broadly in a society. When a cyberattack occur, it can shut down AI systems that people rely on. For instance, a healthcare diagnostic tool or an automated financial service. This situation represents the disruption as immediate damage. However, the long-term damage is often worse. When people learn their data was exposed or that an AI system was easily manipulated, they may become sceptical of the entire technology.
As a result, one can say that digital trust is asymmetric. It takes a long time to build but can be destroyed instantly. Even if an organisation has done everything "by the book" ethically speaking and has a robust AI governance internal framework, a single security incident can undo lots of work building digital trust. Let’s take an AI system used for job applications that is compromised and reveals sensitive personal information of thousands of applicants. People won't care if the system was designed with ethical principles in mind. The breach itself becomes the dominant narrative.
This is why cybersecurity isn't just a technical requirement for AI systems, CISOs play a vital role in maintaining the digital trust that allows these AI technologies to exist in our society at all.
Integrating Cybersecurity into AI Governance Frameworks
A crucial takeaway is that cybersecurity must be a foundational pillar of AI governance to ensure system reliability. Neglecting cybersecurity in the early stages of AI governance risks undermining even the most ethically sound AI designs. My main argument is that cybersecurity protocols not only protect against threats but ensure that AI systems function as intended, thus reinforcing their ethical and regulatory foundations. For example, by instituting robust data validation and authentication measures, cybersecurity experts contribute directly to reducing algorithmic bias and ensuring that AI models remain accountable to their intended purposes.
In that context, CISOs evolve beyond their traditional threat mitigation role to become strategic partners in AI development and deployment. This evolution demands that CISOs develop expertise in AI-specific vulnerabilities, from adversarial attacks that compromise model integrity to data poisoning that undermines training sets. The CISO must collaborate with data scientists to implement continuous security validation throughout the AI lifecycle, from conception to decommissioning.
CISOs are increasingly responsible for establishing threat modeling frameworks specifically tailored to AI systems, identifying unique attack surfaces that conventional cybersecurity approaches might miss. They must pioneer new security metrics that can measure the robustness of AI models against manipulation and the integrity of data pipelines that feed these systems. As AI governance frameworks mature, CISOs should lead the development of incident response protocols designed for the unique challenges posed by compromised AI systems, where impacts can cascade rapidly through automated decision chains.
Additionally, the CISO's role extends to operationalizing regulatory compliance within AI systems. As regulations like the EU AI Act or South Korea's AI Basic Act emerge globally, CISOs would start translating abstract compliance requirements into concrete security controls.
In that sense, a collaborative approach is required where cross-functional stakeholders come together to establish standards that safeguard technical and ethical aspects of AI. Organizations must adopt a forward-looking approach that fully integrates cybersecurity in every phase of AI development and operation. The CISO stands at the center of this integration, serving as guardian and enabler of responsible AI deployment. After all, digital trust placed in AI systems rests on our collective ability to anticipate, respond to, and mitigate cyber threats.
Share this
You May Also Like
These Related Stories
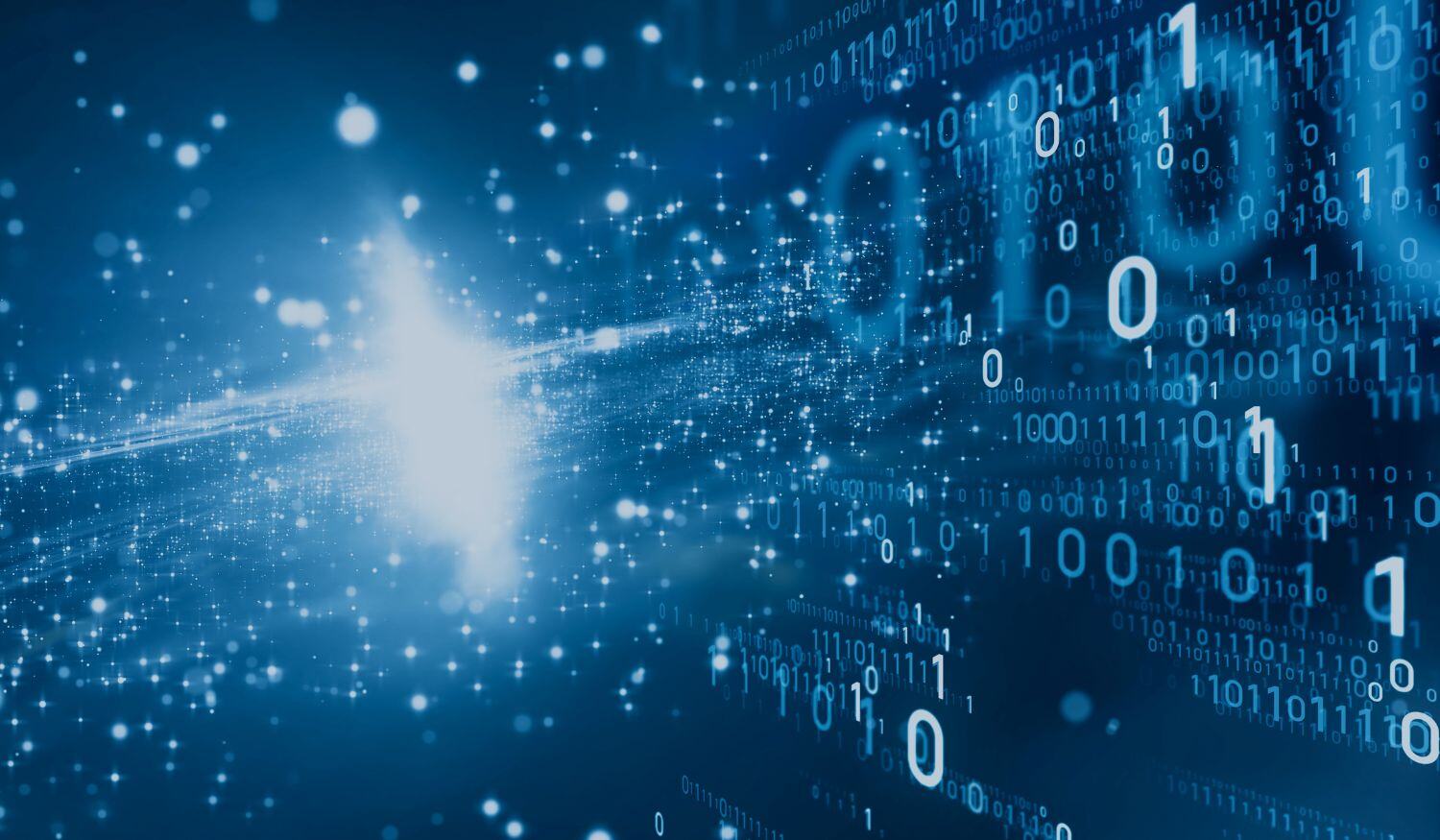
Quantum-Resistant Threat Entropy Index with AI-Driven: Lattice Cryptography
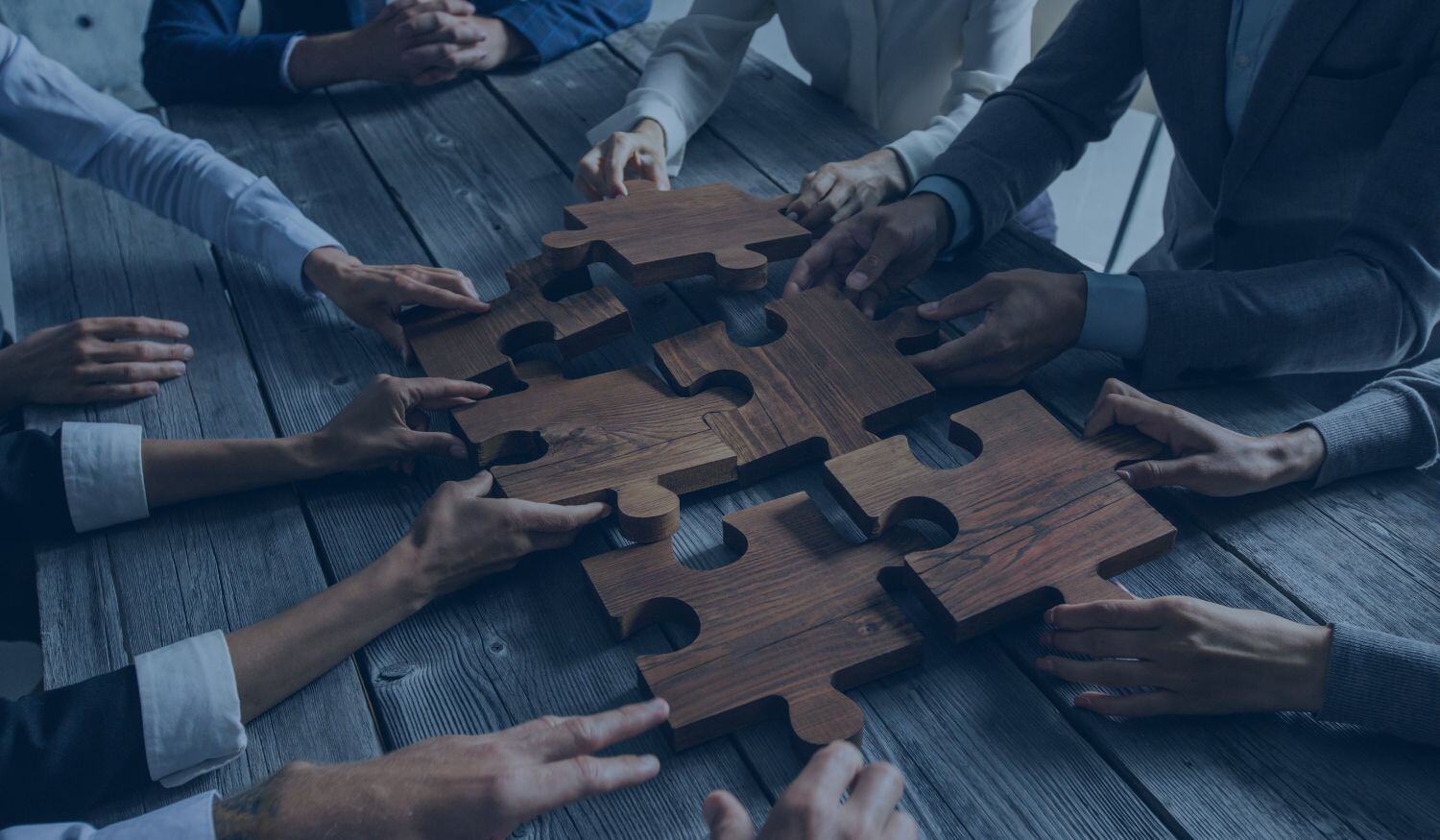
Balancing Cybersecurity and Business Growth Using a Risk-Based Approach
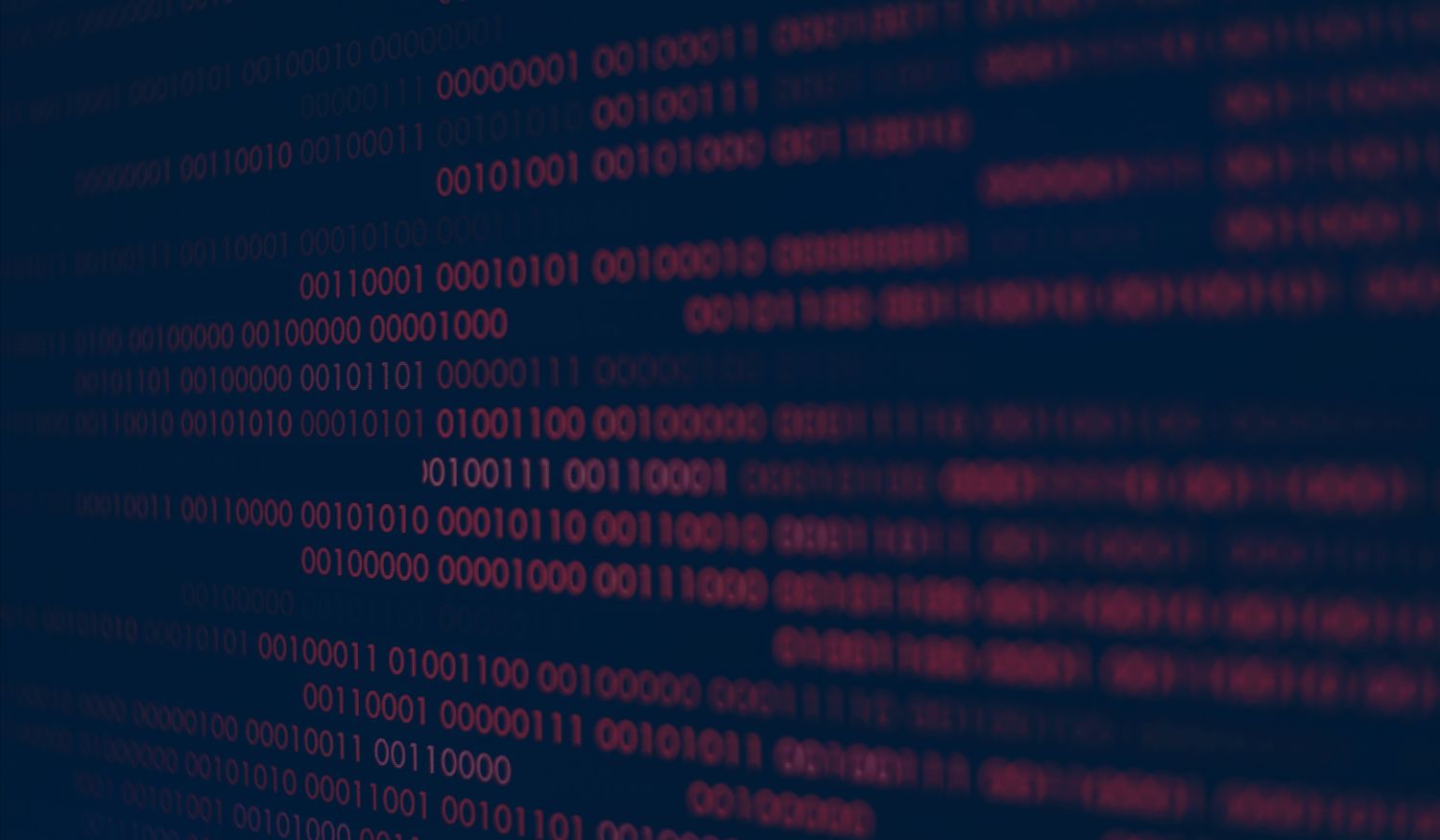