Balancing Generative AI Innovation with Legal and Data Security Concerns
Generative Artificial Intelligence (AI) stands as a pinnacle of innovation, leveraging neural networks to create content mimicking human behavior. From text to images and code, these AI models learn patterns and generate new, often indistinguishable outputs. While hailed for its potential, Generative AI introduces a new frontier of legal and data security challenges. This article explores the benefits and risks of Generative AI within Cybersecurity before providing tips on how software developers can mitigate these risks.
Generative AI Innovation in Cybersecurity
Generative AI provides significant advancements to enhance an organization's cybersecurity efforts. Its ability to predict and identify potential threats, simulate attacks, and generate secure code snippets aids in fortifying defenses. This technology allows developers to simulate diverse attack scenarios, enabling proactive security measures. Additionally, AI-generated code can augment existing security frameworks by offering innovative solutions and robustness in defense mechanisms. However, many have raised signification concerns that come alongside these advantages. Two industry experts recently highlighted the power of generative AI and you can find out in more detail how it has aided cybersecurity in a recent Fireside chat hosted by Cyber Security Tribe.
5 Legal and Cybersecurity Risks of Generative AI
Despite its promise, Generative AI poses distinct legal and security risks, causing concern within software development circles:
- Open-Source license violation
- Copyright law violation
- Security vulnerabilities exposure
- Proprietary information disclosure
- Exposure of sensitive data
Open Source and Copyright Concerns
Generative AI's reliance on public code repositories, such as GitHub, blurs the lines of intellectual property rights and open-source licensing. The inherent nature of these models, learning from and potentially reproducing snippets from publicly available code, raises concerns about compliance with licensing agreements. The challenge lies in ensuring that AI-generated outputs properly credit original authors and adhere to the terms outlined in open-source licenses. This requires a nuanced approach, balancing innovation while respecting the intellectual property of contributors to the code repositories.
Security Breach Vulnerabilities
The opacity of AI-generated code complicates the identification and mitigation of security vulnerabilities. Unlike human-written code, AI-generated outputs lack clear, understandable logic, making it challenging to trace potential weaknesses. This opacity increases the risk landscape, necessitating stringent scrutiny and comprehensive testing methodologies. Traditional security assessment techniques might prove insufficient, demanding innovative approaches to analyze and fortify AI-generated code against potential threats. Continuous monitoring, robust testing protocols, and collaboration between AI experts and cybersecurity professionals become imperative to detect and address vulnerabilities promptly.
Safeguarding Proprietary Data
Protecting proprietary information inadvertently embedded within AI-generated code becomes crucial to safeguard an organization's competitive advantage and brand integrity. As AI models learn from a vast array of data, there's a risk of sensitive company-specific information becoming unintentionally embedded. Implementing robust data governance frameworks, encryption methods, and access controls becomes essential to prevent inadvertent leaks. Moreover, organizations must institute stringent policies regarding the use and handling of proprietary information within AI training sets to mitigate the risk of exposure.
Mitigating Data Exposure
The inadvertent exposure of sensitive data within AI-generated outputs poses significant challenges to data privacy and regulatory compliance. To mitigate this risk, organizations need meticulous data governance measures. This includes implementing encryption techniques, pseudonymization, and anonymization protocols to protect sensitive information embedded within AI-generated outputs. Moreover, regular audits, strict access controls, and continuous monitoring are imperative to identify and rectify potential data leaks, ensuring compliance with stringent data protection laws and regulations.
How Software Developers Can Mitigate the Risk of Generative AI
As the integration of AI like ChatGPT and Copilot revolutionizes software development, developers must prioritize safety measures to harness their benefits without compromising privacy or security.
Awareness of Privacy Risks
AI solutions like ChatGPT entail the collection of personal data from chat sessions, presenting inherent privacy risks. Developers leveraging these tools need to exercise caution regarding the information shared within these interactions. Recognizing the potential for sensitive data exposure prompts developers to implement protective measures proactively.
Mitigating Privacy Risks
Tools like SafeType, an open-source extension developed by Cyera Labs for Chrome and Edge browsers actively alerts users during ChatGPT sessions when inputting sensitive data and empowers them to automatically anonymize this information. Such measures help mitigate privacy risks, enhancing data protection during AI-driven development processes.
Implement Comprehensive Data Governance
Developers should establish robust data governance frameworks tailored to AI utilization. This involves implementing encryption, anonymization, and pseudonymization techniques to safeguard sensitive information shared or generated during AI-powered interactions. Regular audits, access controls, and monitoring mechanisms further fortify data protection protocols.
Conclusion
Generative AI brings unparalleled innovation alongside intricate legal and security challenges. While bolstering cybersecurity, it also poses risks like open-source violations and data exposure. Safeguarding against these threats demands vigilant data governance and proactive privacy measures. Developers must navigate this landscape with caution, leveraging tools like SafeType to mitigate privacy risks. As the AI landscape evolves, a commitment to ethical deployment and continuous vigilance will be crucial in harnessing Generative AI's potential while safeguarding data integrity and privacy.
Share this
You May Also Like
These Related Stories
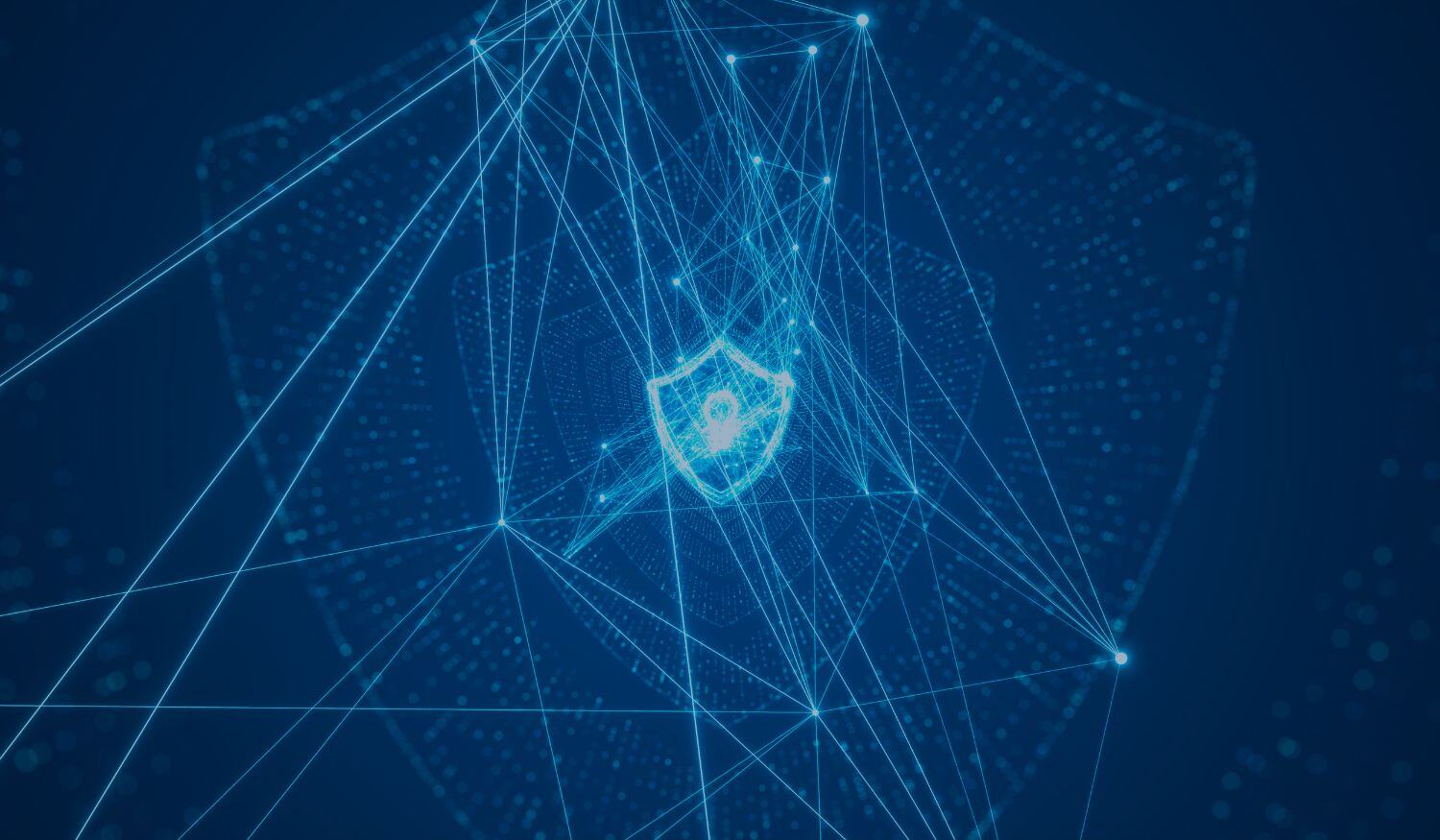
AI is a Hidden Risk to Organizations
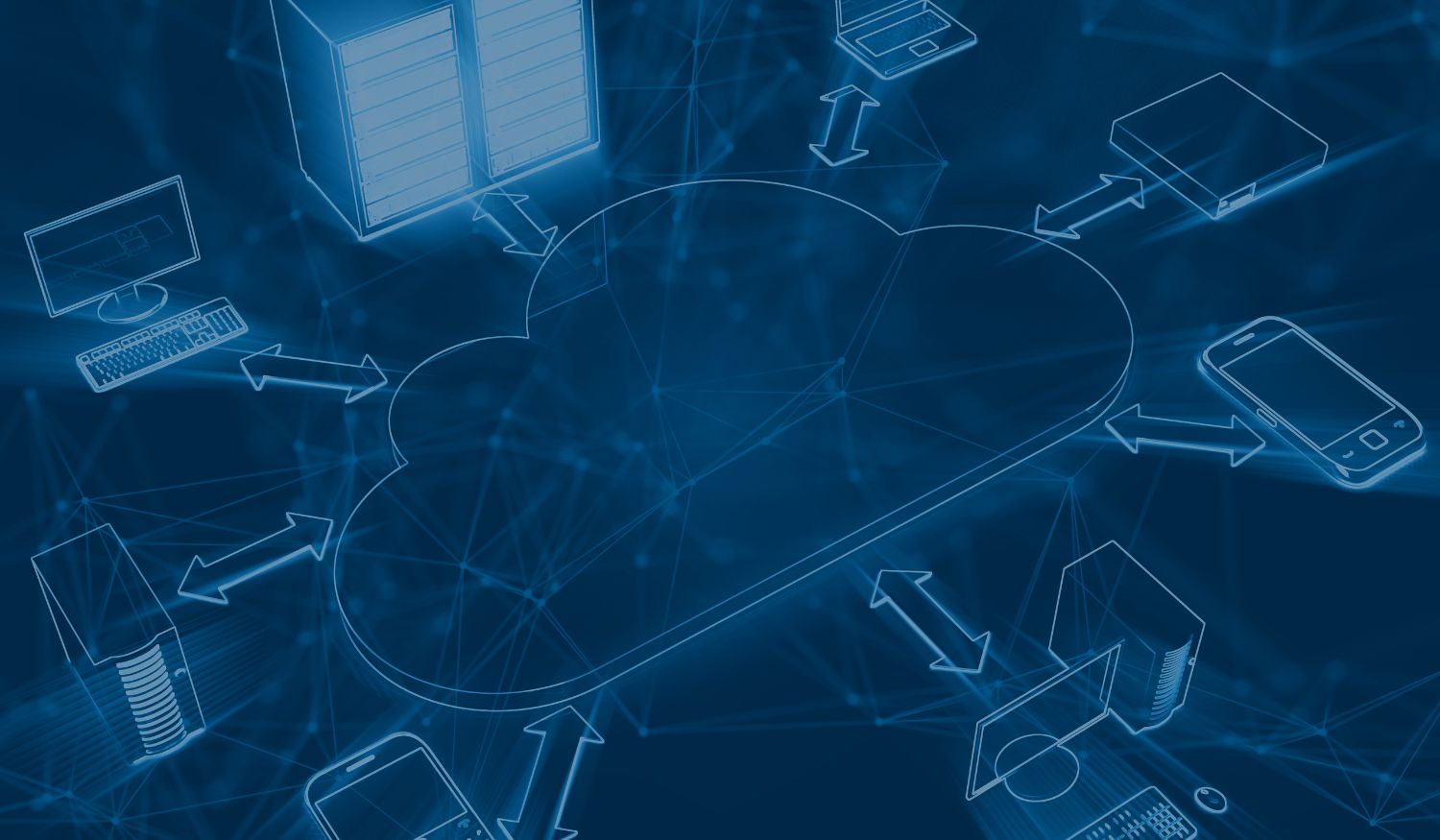
Navigating the Cloud Data Security Maze in 2024
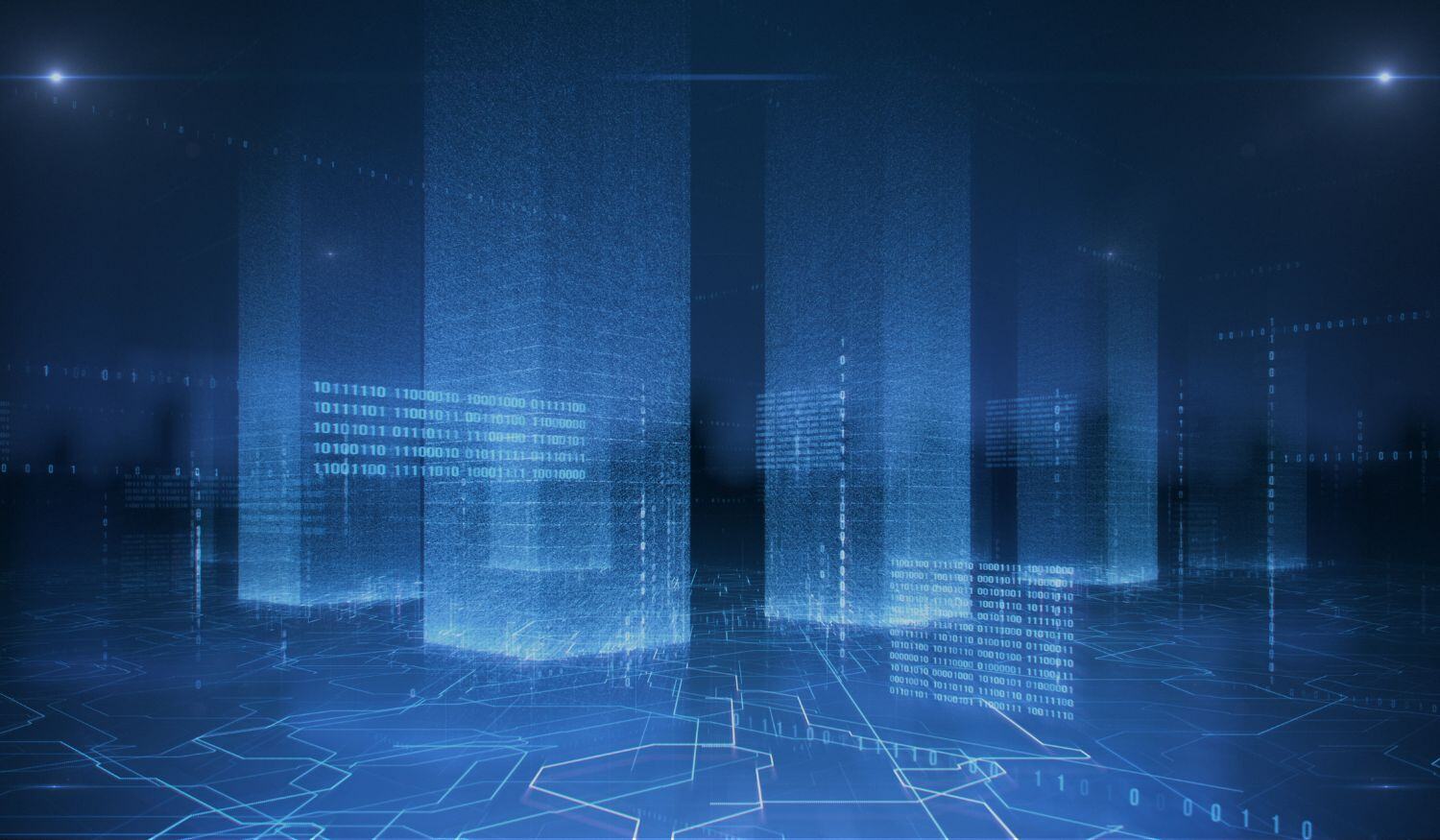