How AI has Transformed Cybersecurity in 2024
2024 has seen the continuation of artificial intelligence (AI) playing a transformative role, both for attackers and defenders within the cybersecurity market. Cyber Security Tribe interviewed four senior tech leaders at InfoSec Europe 2024 in London regarding the use of AI, specifically asking how threat actors are utilizing AI to breach defenses in 2024 and conversely how organizations are deploying AI to help mitigate these attacks.
Matt Aldridge of BrightCloud at OpenText Cybersecurity highlights how AI streamlines sophisticated cyberattacks, such as automated phishing and rapid exploit development. Christopher Doman from Cado Security discusses AI’s use in coding defense mechanisms and analyzing attack data. Dave Merkel of Expel focuses on AI's potential to enhance detection and operational efficiency, while Andy Mills of Cequence explores the role of AI in identifying and mitigating API-based threats. Through these insights, the article explores how AI is revolutionizing cybersecurity and strengthening cyber defenses while making attacks more formidable.
Matt Aldridge, Principal Solutions Consultant & Cyber Evangelist, BrightCloud at OpenText Cybersecurity
AI is transforming cybersecurity, enabling both defensive and offensive strategies. On the offensive side, AI significantly enhances targeted attacks like spear phishing. Previously, attackers had to manually select and research targets for susceptibility to social engineering. Now, AI can automate this process, identifying likely victims and crafting convincing phishing lures. This lowers the barrier to entry, allowing even less sophisticated actors to launch effective, lucrative campaigns.
AI also accelerates exploit development. Traditionally, exploiting software vulnerabilities required high expertise to interpret and exploit CVEs (Common Vulnerabilities and Exposures). Now, AI models can generate exploit code directly from CVEs, speeding up the process and enabling more actors to engage in exploit development, leading to increased data theft and ransomware attacks.
Once inside a system, AI helps criminals manage stolen data, often unstructured and vast. AI tools can sift through this data, identifying valuable information like credentials or financial data quickly. This repurposing of generative AI, originally designed for benign tasks, facilitates malicious activities.
Another emerging threat involves adversarial AI attacks, where AI models themselves are targeted. Designers must now embed controls to prevent misuse and defend against adversarial techniques that could extract unintended functionalities or confidential data from AI models.
On the defensive side, AI has been integral for years. It helps classify URLs, identify malware, and detect anomalies in user behavior. AI tools classify web content to gauge cybersecurity and legal risks, and unsupervised machine learning aids in anomaly detection for user and entity behavior analytics. This helps identify unusual activities that may indicate malicious behavior or compromised systems.
In Security Operations Centers (SOCs), generative AI supports analysts by simplifying threat data queries and processing large data volumes to pinpoint vulnerabilities. This reduces manual effort, allowing analysts to focus on critical threats and optimize cybersecurity defenses. Overall, AI is essential in scaling human resources to manage the expanding threat landscape and protect against sophisticated cyber threats.
Christopher Doman - Co-Founder/CTO at Cado Security
The rise of AI-generated malware, initially feared, hasn't materialized significantly in real-world attacks. There were early demonstrations, such as Worm GPT, which scanned the internet with moderate success, but these haven't led to highly damaging, widespread attacks. However, AI has improved phishing attempts, making them more convincing, and attackers have leveraged AI to assist in code writing, although concrete evidence of this is limited due to the clandestine nature of attackers' development environments.
Interestingly, some unconventional uses of AI have emerged, particularly in gaming. In Russian-speaking regions, game hacks utilizing OpenAI APIs have been developed to automate in-game actions like mining gold and impersonating players, highlighting how AI can be used creatively for dubious purposes.
From a defense perspective, large language models and other AI techniques have proven beneficial. AI helps developers write code to defend against attacks, create detections, and analyze data post-attack. However, due to the sensitivity of the data involved—such as full disk images and credit card information—using external AI services like OpenAI isn't feasible. Instead, localized models in custom environments are used to ensure data security, automating the reporting and analysis of incidents while keeping human verification in the loop.
AI is also being used effectively for proactive and responsive security advice. It scans extensive documentation to identify and correct security misconfigurations, making it a valuable tool for maintaining robust cybersecurity practices. Despite these advances, the role of AI in cybersecurity remains largely supportive, augmenting human efforts rather than replacing them entirely.
Dave Merkel, Co-founder and Chief Executive Officer at Expel
AI, especially generative AI, significantly enhances the quality and efficiency of cyberattacks. It excels at mimicking human language, transforming poorly crafted phishing emails or texts into convincing, fluent messages. This eliminates the need for attackers to possess strong language skills, allowing non-English speakers to launch effective attacks against English-speaking victims. Consequently, the pool of potential attackers widens, and the quality of phishing attacks improves.
Research has explored the possibility of AI-generated malicious emails attacking AI-based defenses in an automated back-and-forth battle, but this remains largely theoretical. There has been no concrete evidence of real-world “in the wild” examples of fully automated AI offensive versus defensive scenarios.
On the defensive side, companies are integrating AI to enhance cybersecurity measures. AI is being used to augment existing detection frameworks, write reports, and for communications, and there are obvious places to plug-in this functionality to create efficiency gains. For instance, maybe AI improves the efficiency of robots handling human communication, going from, say, 30% to 60% automation (as an example). This could also extend to co-piloting technologies, where perhaps AI helps security operations analysts make better decisions, boosting their efficiency significantly.
Cybersecurity vendors are also researching how AI can enhance detection capabilities by identifying specific properties of large language models (LLMs) that excel at detecting particular types of attacks. This could lead to discovering previously undetectable threats or improving the efficiency of threat detection. The ultimate goal is to make Security Operations Centers (SOCs) more efficient, requiring fewer analysts to manage a growing number of customers while maintaining high-quality service. This improves the gross margin and operational effectiveness.
While the future applications of AI in cybersecurity are still being explored, current implementations already demonstrate significant benefits in improving the efficiency and quality of cyber capabilities.
Andy Mills, VP of EMEA for Cequence
Detecting Application Programming Interfaces (APIs) attacks is already challenging because these seek to subvert the way an API operates. By discovering and studying the blueprint of the API, the attacker is able to utilise it by sending a request to initiate a process ie to obtain access to sensitive date. As it’s a legitimate request, it’s unlikely to trigger an alert unless the volume of requests is higher than expected. In fact, many organisations base their entire defence on addressing volumetric attacks but in so doing miss low level insidious attacks. It’s this attack paradigm that could see Generative AI become a game changer as it could see those missed attacks grow in number.
GenerativeAI (GenAI) will allow threat actors to make their attacks more targeted, thereby reducing noise on the network, and more evasive, by pivoting through tactics, techniques and procedures (TTPs). That means attacks are going to become much harder to both detect and defend against. Defence mechanisms such as the web application firewall (WAF) will still have a part to play but they must be complemented by real-time behaviour-based threat detection which takes into consideration other indicators such as IP address, User-Agent, and Headers etc. Fingerprint detection will become crucial as it sees these factors used to identify anomalous or malicious traffic patterns, before using these to generate rules, models, and policies for attack mitigation.
GenAI will take the fingerprinting concept a stage further, however, to the point where it is automated to rapidly respond to changing TTPs. Threat finger printing will no longer require those client indicators to discern an attack which means the threat actor doesn’t know which indicator is giving them away, what they need to rotate, when and why. Moreover, defenders will be able to use GenAI to create fake API responses as the attack plays out, further obfuscating the situation. And we will see the emergence of parallel threat hunting across multiple API endpoints so that these fingerprints and threat patterns are used concurrently. But GenAI will also see a more holistic approach taken to API security, with a focus on the entire API lifecycle. Preproduction APIs will have customised security testing with flexible authentication profiles and adaptive test cases, allowing teams to build out thousands of test cases using a combination of source information and learned attacks. Production APIs will use real-time discovery, detection and threat fingerprinting response. And GenAI will facilitate customised runtime discovery with APIs defined by their specific usage and API hosts of interest, ie particular product teams or those hosting AI applications, which will streamline threat detection and response.
Share this
You May Also Like
These Related Stories
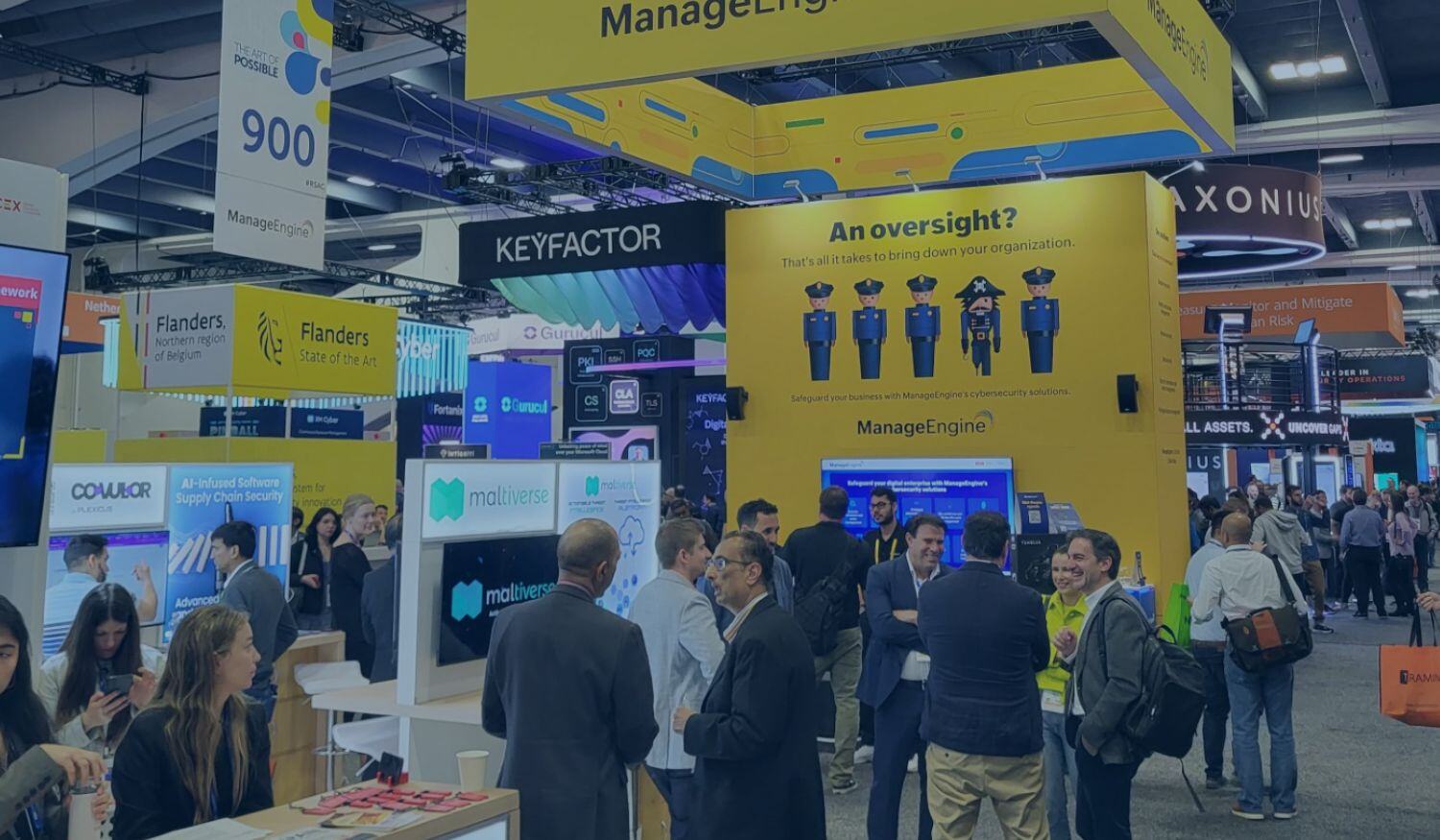
Exclusive AI Insights from Tech Leaders at RSA Conference 2024
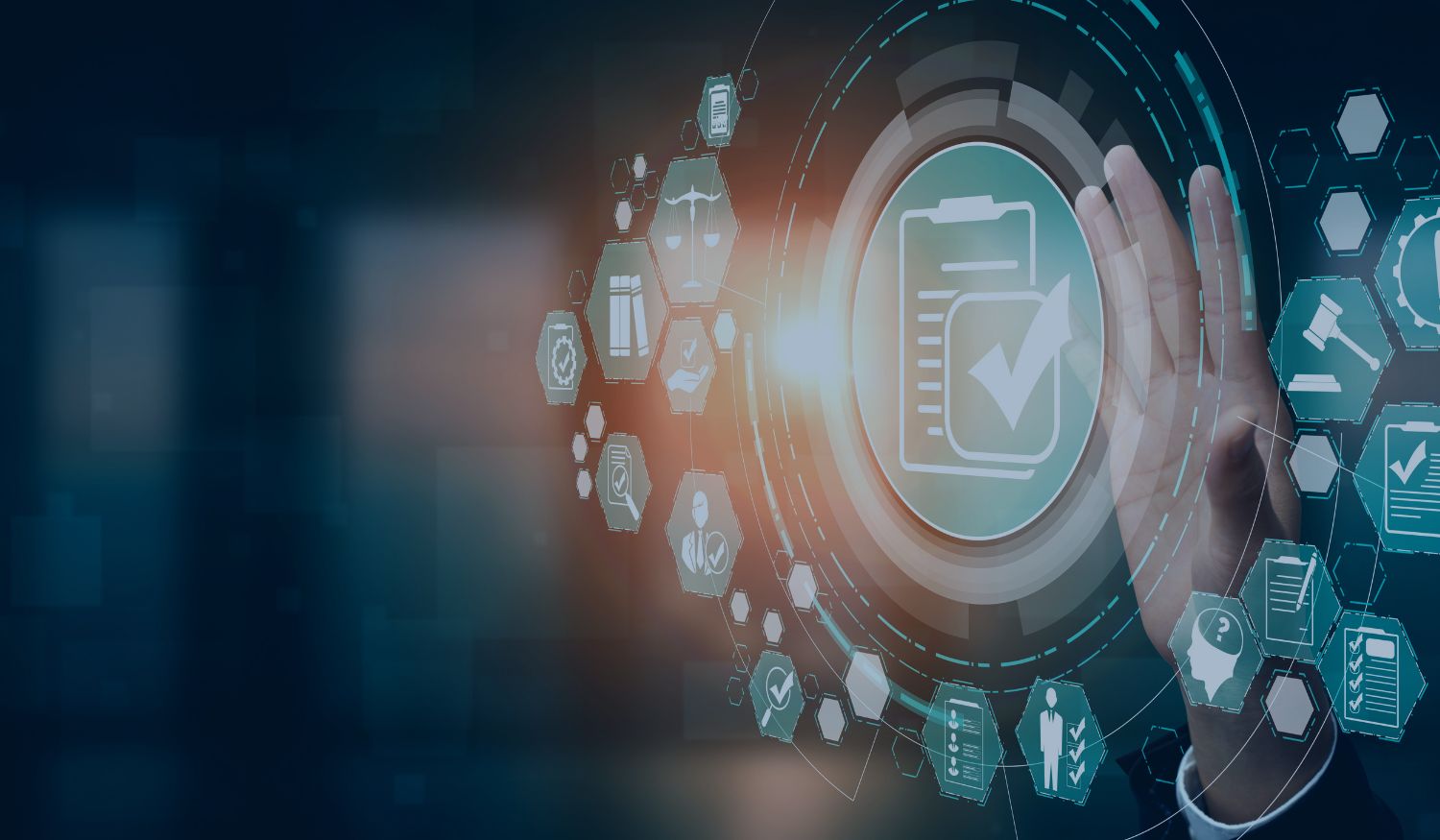
Aligning AI Governance Policies with Organizational Objectives
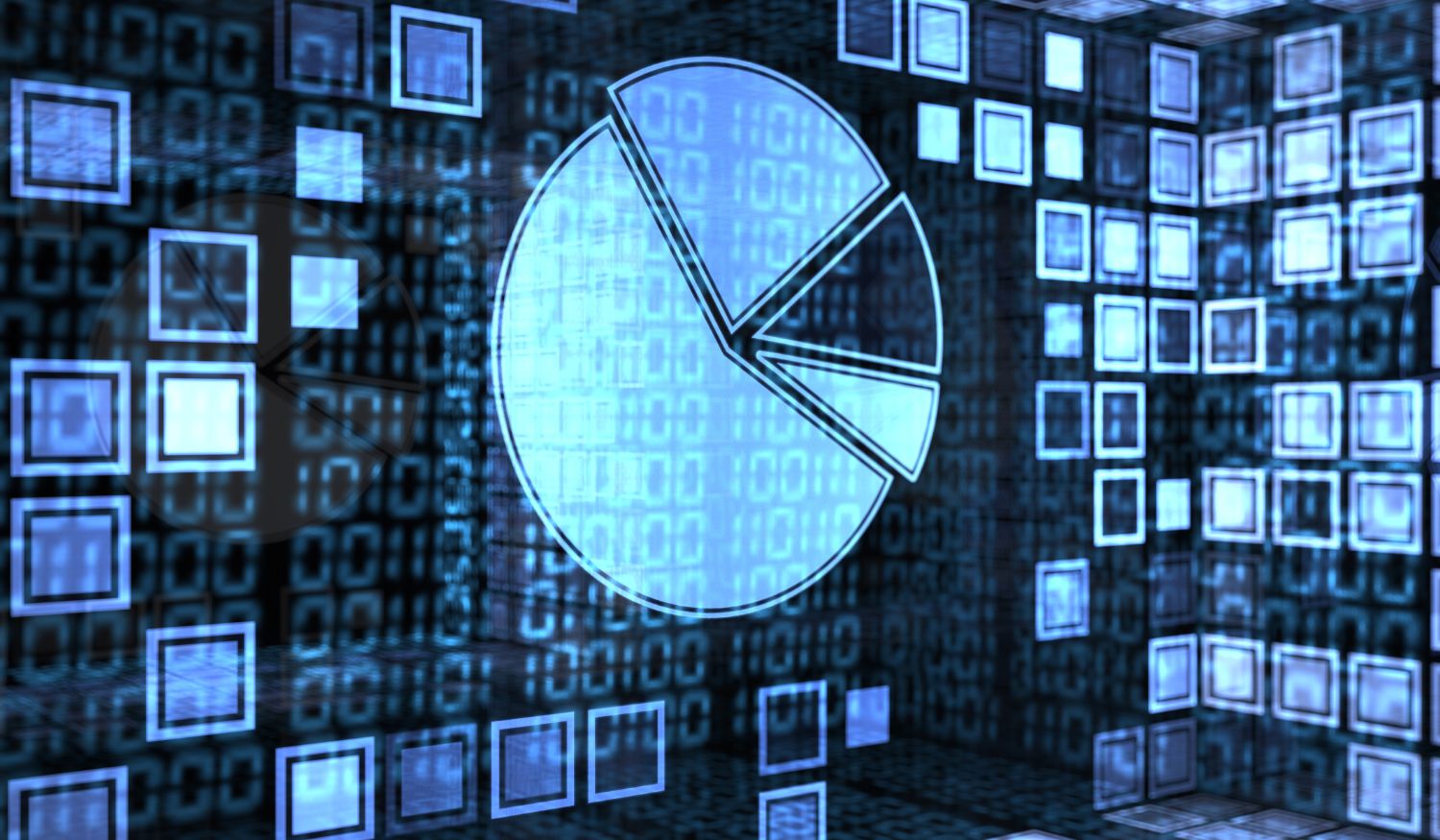