Unlocking the Benefits of Causal AI in Cybersecurity
Traditional artificial intelligence (AI) and machine learning (ML) have widely been incorporated into organizations' cybersecurity defenses, significantly helping analysts and cybersecurity executives find trends and patterns in large datasets at a much faster pace, helping them against the growing utilization of advanced technologies by cybercriminals to breach their defenses.
However, due to limitations of correlation-based machine learning, such as the time-consuming process of building machine learning models, inability to accurately predict future attacks and their lack of adaptability to the ever-changing scaling of services in response to business needs, organizations are recognizing the need for a better approach to AI in cloud environments.
Cyber Security Tribe Advisory Board member Dr. Vivian Lyon considers it a significant problem that cybersecurity executives will face in 2024: “My biggest concern in cyber security, as I look toward 2024, is that some AI-driven security operations centers (SOCs) may not be fully ready to detect cyber-attacks and uncover potential threats in real-time as effectively and efficiently as the attackers that exploit their machine learning (ML) algorithms.”
Darko Matovski, Co-Founder and CEO at causaLens, states: "The key problem current machine learning systems face is that, when it comes to predicting the future, correlations are inadequate. The correlations that have held in the past may simply not continue to hold in the future. Moreover, because correlations are just single numbers, they are not well suited to capturing complex real-world relationships and context."
Causal AI has emerged as an exciting development to aid an organization's defenses helping overcome these these issues and this article explores the role of Causal AI in cybersecurity and four benefits when it is implemented into an organization's defenses.
Understanding Causal AI and its Role in Cybersecurity
Causal AI, also known as Causality AI, is a rapidly evolving field that leverages advanced machine learning algorithms to understand and analyze cause-and-effect relationships within complex systems. It is widely believed to make a significant impact on protecting the future of AI/ML systems.
In recent years there has been a surge of research interest in Causal AI, with major tech giants such as Microsoft, Amazon and Facebook making substantial investments. According to industry projections, the Causal AI market is poised for remarkable growth, with estimates suggesting a substantial increase from USD 8 million in 2023 to an impressive USD 120 million by 2030.
In the context of cybersecurity, it plays a crucial role in identifying the underlying causes of cyber threats and attacks, enabling organizations to develop proactive defense strategies.
Studies and research released in publications regarding its use within cybersecurity appear as early as 2012 (figure 6, Causality and Machine Learning Review), however the availability of increased computational power has greatly amplified the potential of Causal AI within cybersecurity in recent years.
By analyzing vast amounts of data and identifying causal relationships, Causal AI helps security professionals gain a deeper understanding of how cyber threats originate and spread. This understanding allows them to identify vulnerabilities and potential attack vectors, leading to more effective threat detection and prevention measures.
Moreover, it helps in determining the impact of different security controls and measures on the overall cybersecurity posture. By understanding the causal relationships between security actions and their outcomes, organizations can optimize their defense strategies and allocate resources more efficiently.
4 Benefits of Causal AI in Cybersecurity
- Improved Threat Detection and Prediction: Causal AI can help cybersecurity systems better understand the causal relationships between events and identify potential threats and vulnerabilities. By recognizing the root causes of security incidents, it can predict and detect emerging threats before they escalate, allowing for proactive threat mitigation.
- Reduced False Positives: Traditional AI-based cybersecurity systems often generate false positives, leading to alert fatigue for security analysts. Causal AI can help reduce false alarms by distinguishing between coincidental events and causally connected events, resulting in more accurate and actionable alerts.
- Effective Resource Allocation: Causal AI can assist in the efficient allocation of cybersecurity resources. By identifying the causal factors behind security incidents and understanding which components are most critical to the organization's operations, it can help prioritize resource allocation. This ensures that resources such as personnel, budget, and time are allocated where they are most needed, optimizing the overall cybersecurity strategy and resource management.
- Adaptive Defense Strategies: Causal AI can adapt its defense strategies based on the changing threat landscape. It can assess how changes in the network or system may affect cybersecurity and make adjustments accordingly. This adaptive nature enables cybersecurity systems to stay ahead of evolving threats.
Causal AI offers a range of benefits in the field of cybersecurity. From enhanced threat detection and proactive prevention to risk mitigation, reduced false positives, improved resource allocation, its adaptive nature, and reducing attack surfaces. This advancement in AI/ML can help organizations to stay ahead of cybercriminals and protect their critical assets.
As the cybersecurity landscape becomes increasingly complex, embracing Causal AI becomes imperative for organizations to enhance their security measures and ensure a robust defense against evolving threats.
Share this
You May Also Like
These Related Stories
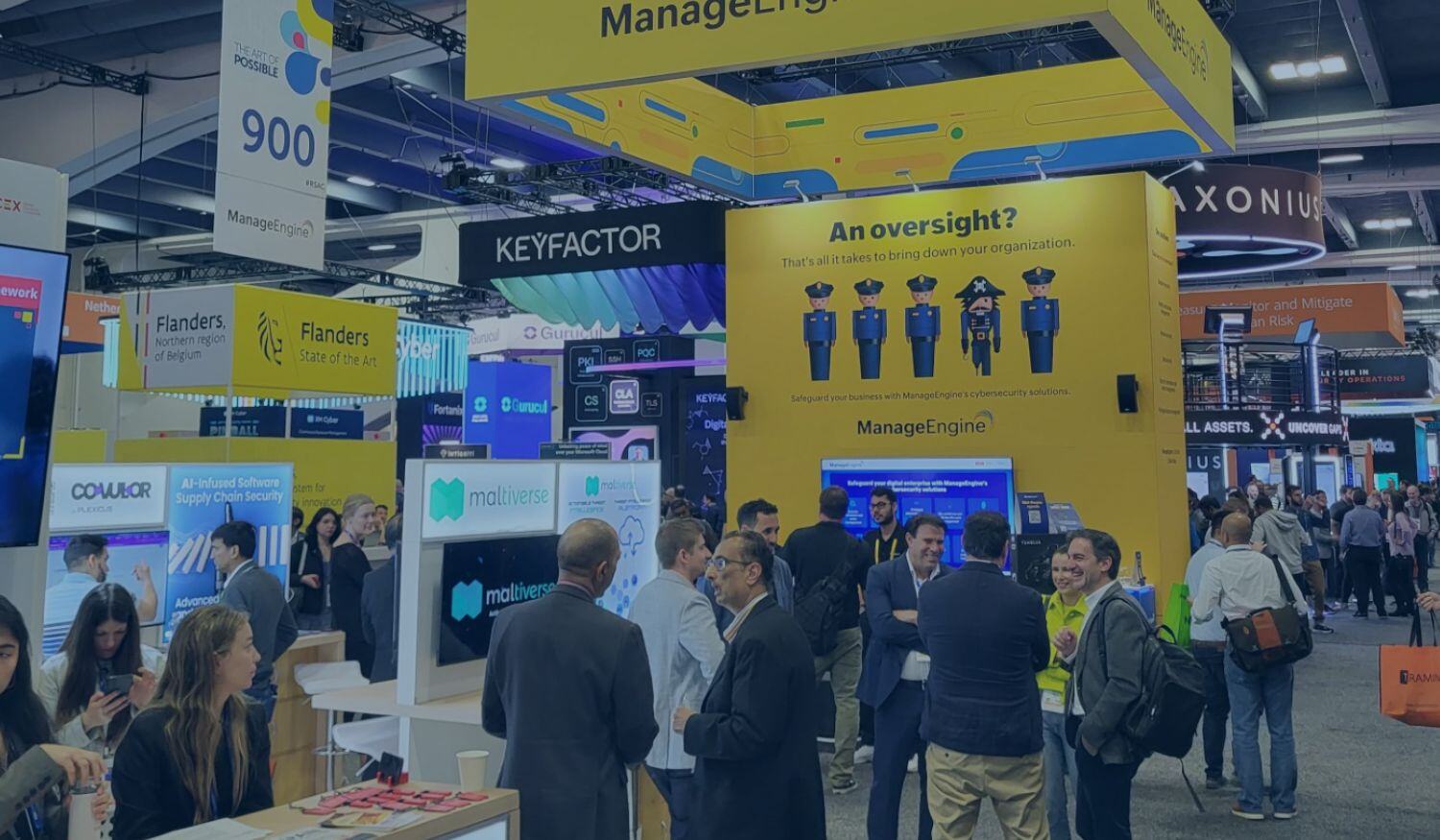
Exclusive AI Insights from Tech Leaders at RSA Conference 2024
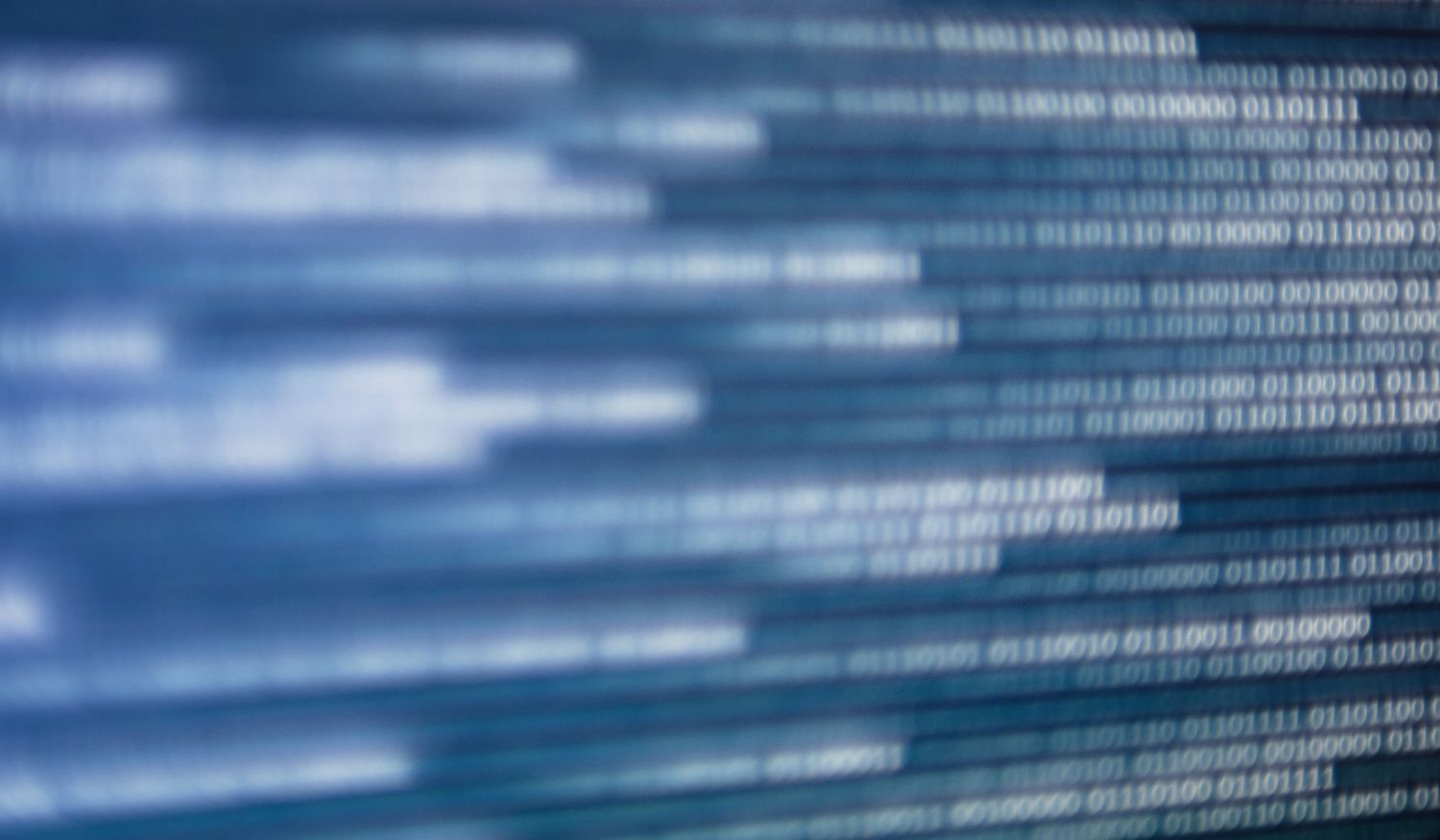
5 Tips to Mitigate the Risk of Sensitive Data Exposure
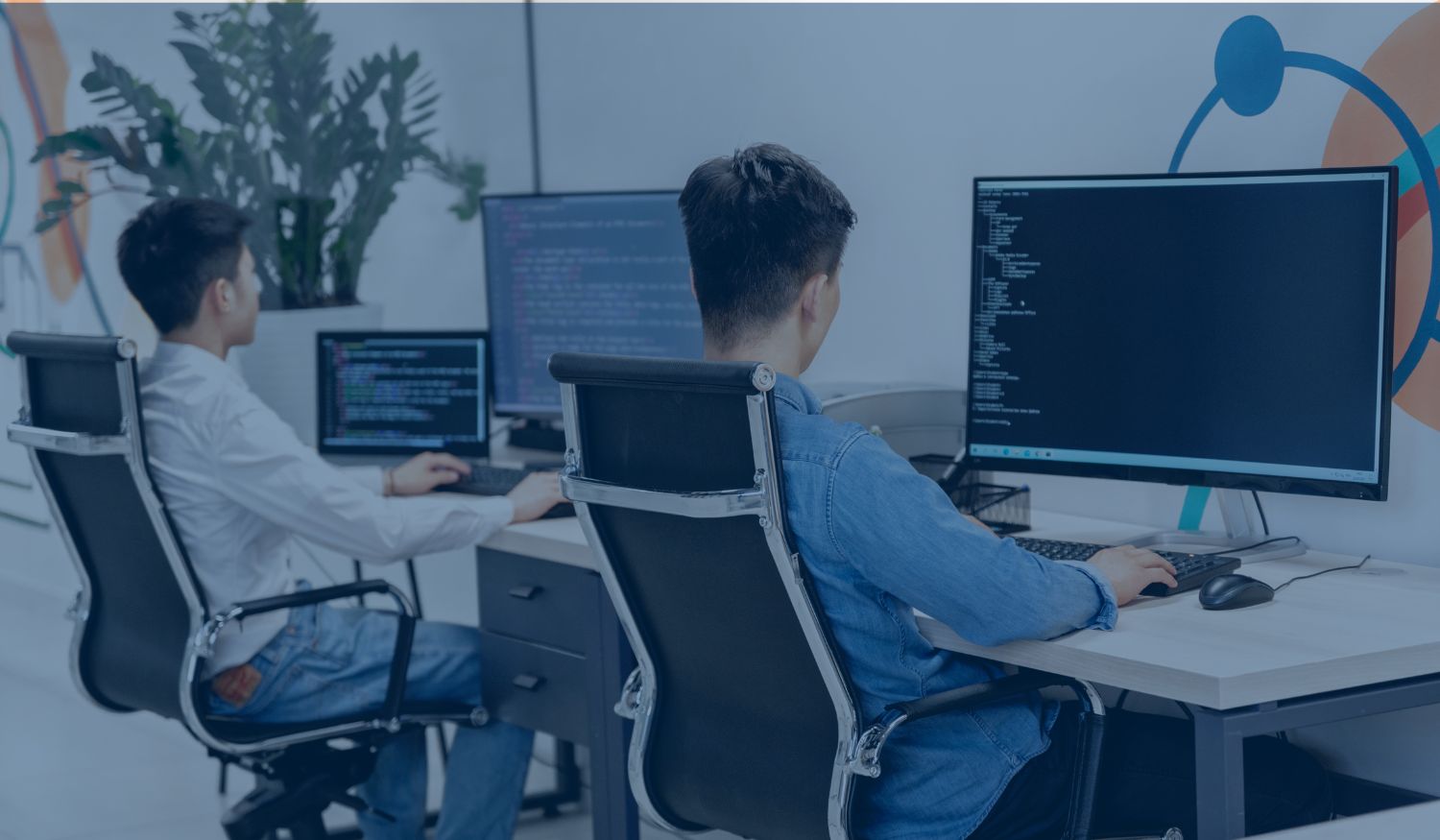